The Economic Strain Behind the AI Curtain: Why Financial Sustainability Eludes LLM Providers
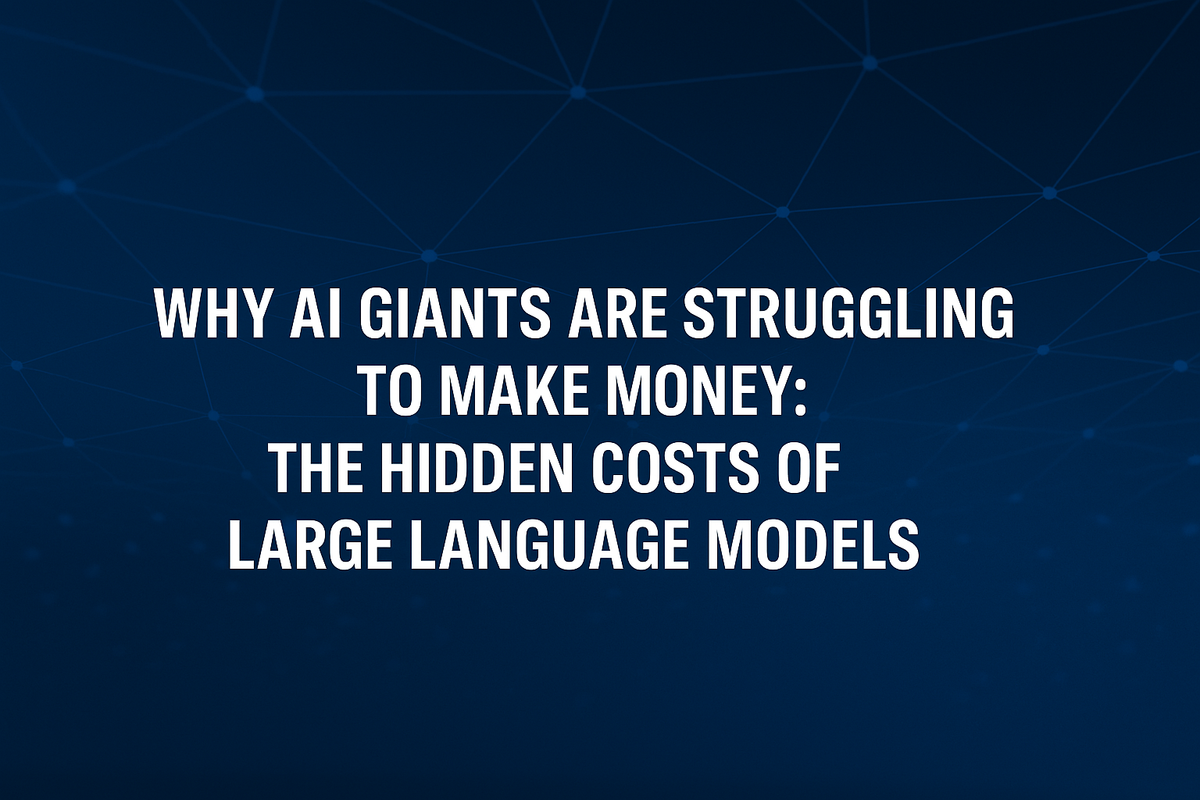
The generative artificial intelligence (AI) revolution, catalyzed by the widespread deployment of large language models (LLMs), has been one of the most transformative technological phenomena of the 21st century. Since OpenAI's release of ChatGPT in late 2022, a cascade of innovation has swept across the AI ecosystem, with both established tech giants and emerging startups racing to build, fine-tune, and deploy increasingly powerful models. LLMs have found applications in diverse fields—from creative writing, programming, and customer service to legal analysis and enterprise productivity. Their potential has been heralded as revolutionary, promising to reshape industries, displace outdated workflows, and enable new forms of human-computer interaction.
The excitement has translated into a flood of capital. Venture firms, institutional investors, and strategic corporate backers have poured billions of dollars into LLM-centric companies. In parallel, the world’s largest cloud providers have reoriented their infrastructure and software stacks around AI, building supercomputers and crafting dedicated service offerings. The rapid evolution of model architectures—from GPT and PaLM to Claude, Gemini, and Mixtral—has turned AI development into a high-stakes arms race.
However, behind the headline-grabbing valuations and product demos lies a less glamorous, but increasingly urgent, reality: the economics of running and maintaining LLMs are daunting. Despite the promise of scalability and ubiquitous automation, most providers are now grappling with significant financial challenges. These include escalating compute costs, diminishing marginal returns on training larger models, and a monetization landscape that is still nascent and often structurally flawed.
The cost to train and run LLMs is not merely high—it is fundamentally different from many previous technology platforms. Unlike traditional software, which can scale efficiently once written, LLMs require continuous capital injection. This is not only due to their voracious appetite for GPU resources during training but also because of the costly nature of inference, particularly when deployed at scale across enterprise and consumer-facing applications. Furthermore, models must be continuously updated to stay relevant, necessitating a persistent research and development (R&D) budget that can stretch even the most well-funded companies.
These structural dynamics have begun to expose a growing fissure in the LLM landscape. While the technological potential is undeniable, the underlying business models remain fragile. A significant number of AI startups are offering models and services at a loss in a bid to capture market share. Many depend heavily on the financial backing of cloud providers that have a vested interest in driving compute consumption. And even among well-known players, revenue growth has not kept pace with operational expenditures. The result is a mounting tension between innovation and sustainability—an issue that could define the next phase of the AI era.
Moreover, market saturation and competitive pressures have begun to erode pricing power. As open-source alternatives continue to improve in performance and efficiency, commercial providers face growing difficulty justifying premium pricing for their APIs and platform solutions. Enterprises, initially excited by generative AI’s potential, are now scrutinizing costs, operational feasibility, and integration complexity. For many, the ROI case remains murky, especially in non-critical use cases where LLMs serve as a convenience rather than a necessity.
This landscape is further complicated by the macroeconomic environment. After a decade of low interest rates and abundant liquidity, the post-2022 financial climate has shifted markedly. Investors are more cautious, capital is more expensive, and the pressure for profitable, sustainable business models is more intense. The tolerance for long burn rates and vague monetization strategies has waned, putting additional pressure on AI startups and incumbents alike to prove their commercial viability.
Against this backdrop, the blog will explore the multifaceted financial challenges faced by large language model providers. We will delve into the cost structure of building and operating these systems, analyze the limitations of current monetization strategies, and assess the broader funding environment. We will also examine how adoption dynamics within enterprises are influencing the revenue potential of LLM services, and what the future may hold for companies navigating this increasingly complex terrain.
By dissecting these core issues, this article aims to provide a comprehensive understanding of why financial sustainability remains elusive for many LLM providers—even as their technical achievements garner global attention. As we will demonstrate, the path to profitability in generative AI is neither straightforward nor assured. It will require a delicate balancing act between continued innovation, prudent financial management, and the development of business models that can withstand competitive and economic pressures.
In the sections that follow, we will provide a detailed examination of the structural and strategic factors contributing to this financial tightrope—and what steps providers might take to build more resilient futures.
The Cost Structure of Building and Running LLMs
As the field of large language models (LLMs) matures, the financial realities underpinning their development and deployment have become increasingly salient. Unlike traditional software products, which often benefit from favorable unit economics and linear cost scaling, LLMs impose a distinctive and complex cost structure on providers. This section dissects the major financial components associated with creating, refining, and maintaining LLMs, highlighting the factors that make such endeavors particularly capital- and resource-intensive.
1. Capital Expenditures (CapEx): Upfront Investment in Model Development
The financial challenges for LLM providers begin at the earliest stage of model development. Capital expenditures—substantial and largely non-recoverable—are incurred before any commercial benefit can be realized. Chief among these are the costs associated with data collection and processing, compute infrastructure, and training cycles.
a. Data Acquisition and Curation
Although data is often assumed to be “free” on the open web, the quality and usability of data for LLM training present substantial hidden costs. Raw data must be filtered, cleaned, deduplicated, and sometimes manually annotated. Proprietary data, which offers greater model differentiation, often requires licensing agreements or partnerships with content providers. Additionally, the legal and regulatory implications of data sourcing—particularly in light of evolving copyright frameworks—necessitate compliance efforts that further inflate expenses.
b. Compute Infrastructure
Training frontier LLMs demands access to state-of-the-art compute infrastructure, particularly GPUs and AI accelerators such as NVIDIA A100/H100, Google TPUs, or custom chips like AWS Trainium. The hardware requirements are vast: training a single model with hundreds of billions of parameters can involve tens of thousands of GPUs operating continuously for weeks or months. Even for well-capitalized organizations, acquiring and maintaining such infrastructure presents substantial financial strain, exacerbated by global shortages of high-performance chips.
Cloud-based alternatives—such as renting GPUs from providers like AWS, Azure, or Google Cloud—offer scalability but not necessarily cost-efficiency. In fact, many startups report cloud bills in the tens of millions of dollars, with inference costs alone sometimes rivaling the cost of training. Building on-premises data centers, while offering long-term savings, entails massive upfront capital outlay and time-intensive buildouts.
c. Model Training and Fine-Tuning
Training costs are not confined to initial model development. Most providers engage in multiple training iterations—base training, reinforcement learning from human feedback (RLHF), instruction tuning, and continual fine-tuning—to improve alignment, safety, and performance. Each cycle involves reusing or replicating parts of the model training pipeline, consuming more compute and increasing overall costs. Moreover, newer techniques like retrieval-augmented generation (RAG) and agentic workflows, while improving utility, require additional computational layers and latency-optimized infrastructure.
2. Operational Expenditures (OpEx): Ongoing Costs of Serving and Evolving LLMs
Even after a model is trained and launched, the cost curve does not flatten. On the contrary, operational expenses rise significantly as the model is scaled to production, integrated into applications, and updated regularly.
a. Inference and Hosting Costs
Serving LLMs—particularly those with large parameter counts—remains expensive. Unlike traditional search engines or APIs, where marginal costs are negligible, every query processed by an LLM incurs a real-time compute cost. This is due to the need to load and execute large models, often across GPU-accelerated infrastructure, for each user prompt.
Inference costs are further magnified when models support high-concurrency applications, real-time outputs, or multimodal inputs. Some providers attempt to offset these costs via model quantization, distillation, or batching strategies, but such optimizations offer only partial relief.
b. Engineering and Research Headcount
Maintaining a competitive LLM operation requires a sizable, specialized workforce. This includes machine learning researchers, data engineers, DevOps professionals, product managers, and safety experts. The competition for AI talent is intense, and top-tier ML researchers command compensation packages on par with elite hedge fund professionals or investment bankers. Startups and established companies alike must invest heavily in retaining top talent, often requiring equity grants and performance bonuses in addition to base salaries.
c. Monitoring, Safety, and Compliance
As public and regulatory scrutiny around AI increases, providers must dedicate significant resources to model oversight. This includes red-teaming, prompt injection testing, hallucination reduction, and content moderation pipelines. Safety auditing systems must be built and maintained, and many jurisdictions are moving toward legal mandates for model transparency and risk classification.
In addition, organizations operating in regulated industries or international markets must invest in compliance systems to satisfy local laws such as the EU AI Act, China’s Generative AI Measures, and anticipated U.S. federal guidelines. The legal overhead and operational adjustments needed to remain compliant can add a nontrivial layer of ongoing expenditure.
3. The Hidden Costs of Rapid Innovation
The rapid pace of technological advancement in the LLM field introduces an additional, often overlooked cost: obsolescence. Models that were state-of-the-art just a year ago can quickly become outdated due to breakthroughs in architecture (e.g., Mixture of Experts), training techniques (e.g., Chain-of-Thought prompting), or benchmarks. Companies are forced to decide between upgrading their models—requiring additional training runs—or risk falling behind competitors. This race to stay relevant ensures that costs remain perpetual rather than episodic.
Furthermore, the AI industry’s tendency toward centralized, compute-intensive model development favors only those providers with sustained access to capital. For others, the inability to continually invest in updated model architectures and infrastructure often results in strategic vulnerability, particularly as customer expectations evolve and the competitive landscape intensifies.
4. Chart: Breakdown of Total Cost of Ownership (TCO)
To illustrate these financial burdens, a visual representation of the Total Cost of Ownership (TCO) for LLM deployment provides useful insight. The following chart breaks down the proportional cost distribution for a typical large-scale LLM provider:
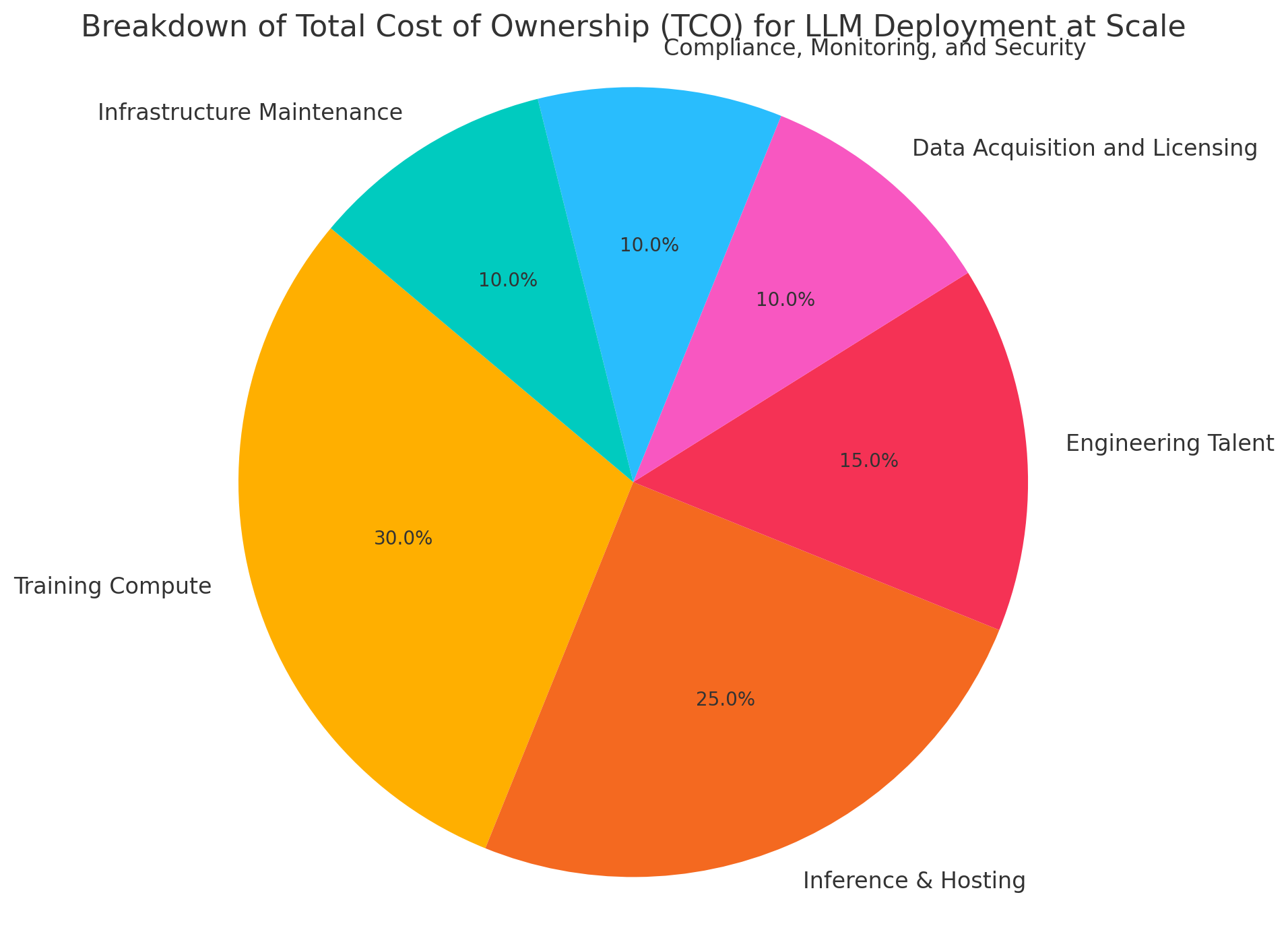
Such a distribution underscores the multifactorial nature of LLM economics. While compute costs are substantial, they are far from the only financial burden; rather, the confluence of recurring costs, talent pressures, and regulatory obligations renders LLM provision a uniquely demanding business model.
Monetization Struggles and Business Model Limitations
Despite the transformative potential and widespread fascination surrounding large language models (LLMs), their monetization remains a formidable challenge. Most providers find themselves navigating an environment where usage is high, but revenues are often insufficient to offset operating costs. The friction between technological capability and economic sustainability is becoming increasingly pronounced, with a growing consensus that the prevailing business models are either structurally flawed or not yet mature enough to justify the immense capital required.
1. The API Economy and Its Constraints
One of the most prevalent approaches to monetizing LLMs has been the provision of application programming interfaces (APIs). In this model, customers pay to access a provider’s model endpoints on a usage-based pricing schedule, typically measured in tokens or characters processed. This model offers simplicity, scalability, and ease of integration, which makes it attractive to developers and enterprises alike.
However, the API-based revenue model is beset with limitations. First, margins are thin. The cost of serving LLM inferences—especially with large models—remains high due to compute requirements. As a result, providers have limited pricing flexibility and often subsidize access to attract and retain customers, hoping to achieve scale that will eventually offset losses. Second, API users frequently churn or reduce usage when faced with cost constraints, leading to volatile and unpredictable revenue streams. Finally, enterprises with significant usage volumes increasingly prefer in-house deployment or fine-tuning open-source models, bypassing API fees entirely in favor of control and cost-efficiency.
2. Freemium-to-Paid Conversion: A Leaky Funnel
Another common monetization approach follows the freemium model, wherein basic access to an LLM-powered tool is offered for free, with premium features gated behind a subscription or usage tier. This strategy, deployed by companies such as OpenAI (ChatGPT Plus) and Google (Gemini Advanced), aims to generate broad user adoption and convert a subset of engaged users into paying customers.
While freemium has driven enormous top-of-funnel user acquisition, conversion rates remain modest. Many users are satisfied with the capabilities offered in free tiers and lack a compelling reason to upgrade. Moreover, competition has increased user expectations for what should be included without charge. The net result is a leaky monetization funnel, where the majority of infrastructure and development costs are borne without corresponding revenue.
Additionally, consumer willingness to pay for AI services is not universal. In some regions and demographic segments, price sensitivity is high. Furthermore, without a clear productivity or economic benefit tied to usage—as is the case with entertainment or casual exploration—monetization potential remains limited.
3. Competitive Pressures and the Race to the Bottom
The monetization dilemma is further compounded by intensifying competition. The proliferation of LLM providers has triggered a pricing war, where vendors undercut each other in a bid to secure market share. This dynamic is most apparent in the API space, where prices have dropped considerably over the past 18 months. In some cases, models are offered at or below cost, supported by strategic investors or infrastructure partners seeking longer-term platform entrenchment.
A significant disruptor in this pricing calculus is the rise of open-source models. Organizations such as Meta (LLaMA), Mistral, and Stability AI have released performant models under permissive licenses, allowing enterprises to host and modify them with minimal restrictions. These models, while not always matching the capabilities of proprietary alternatives, are improving rapidly and offer compelling cost advantages. For many developers and organizations, the marginal performance trade-off is acceptable, particularly when weighed against vendor lock-in and escalating API costs.
This trend exerts downward pressure on commercial pricing, forcing proprietary model providers to continually justify their value proposition through performance, safety, reliability, or ecosystem advantages. However, these differentiators are increasingly difficult to sustain, especially as model commoditization accelerates.
4. Platform Integration: A Double-Edged Sword
Some LLM providers have sought to embed their technology directly into productivity tools, cloud platforms, or enterprise software suites. Microsoft’s integration of OpenAI models into Microsoft 365, GitHub Copilot, and Azure OpenAI Service exemplifies this approach. While such integrations have generated tangible user benefits and expanded addressable markets, the revenue split and strategic control often remain skewed in favor of the platform provider.
For instance, in cloud-provider relationships, LLM companies frequently act as middleware, offering a model layer on top of infrastructure and distribution channels they do not control. This not only reduces pricing power but also constrains long-term margin potential. Moreover, end users often attribute the value to the application layer or platform, not the underlying model provider, making branding and differentiation more difficult.
5. Table: Comparison of Revenue Models Among Leading LLM Providers
To provide context on how various companies are navigating monetization, the following table outlines the dominant revenue strategies used by several notable LLM providers:
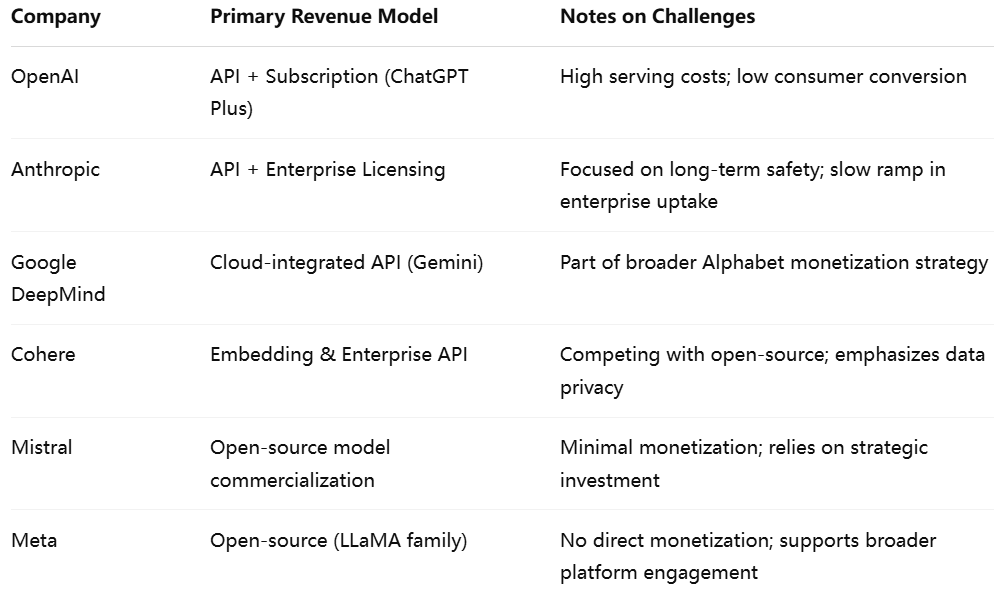
This table illustrates the diversity of approaches and the common challenge of aligning cost-intensive operations with scalable and reliable revenue streams.
6. Evolving Monetization Frontiers
In response to these limitations, providers are exploring new monetization paths. These include:
- Vertical AI solutions: Tailoring LLMs for specific industries (e.g., legal, finance, healthcare) where value can be more directly measured and priced.
- Usage-based agent platforms: Offering AI agents capable of task automation, with pricing aligned to business outcomes or task completion.
- Marketplace ecosystems: Enabling third-party developers to build and sell applications on top of foundational LLM platforms.
While promising, these strategies are still nascent and require significant ecosystem development, trust-building, and operational coordination. Providers must also manage the trade-off between platform openness and monetization control.
Funding Fatigue and the Capital Conundrum
The rapid advancement of large language models (LLMs) has been underwritten by a sustained influx of capital from venture firms, institutional investors, and strategic corporate partners. These investments have fueled an unprecedented wave of innovation and infrastructure buildout, enabling providers to pursue ambitious model development, talent acquisition, and go-to-market strategies. However, as the economic landscape shifts and enthusiasm matures into scrutiny, the funding environment is undergoing a marked transformation. What was once a field awash with capital is now confronting investor caution, demand for sustainable economics, and growing uncertainty about long-term returns.
1. The Era of Hyper-Funding: Accelerated Growth at All Costs
The initial wave of funding for LLM providers was characterized by extraordinary optimism. Investors, eager to participate in what appeared to be a paradigm-shifting technology, engaged in competitive funding rounds at valuations that defied traditional financial metrics. Companies such as OpenAI, Anthropic, Cohere, and Inflection AI raised billions of dollars with limited revenue history, driven by the conviction that foundational AI models would become as indispensable as operating systems or cloud platforms.
This capital was used to accelerate nearly every aspect of business growth—massive GPU purchases, multi-year data center contracts, aggressive hiring of AI research talent, and expansive marketing campaigns. These strategies were not inherently flawed; they were consistent with the “land grab” mentality common to emerging technological platforms. However, they also assumed that monetization would eventually catch up to expenditures—a belief that is increasingly being challenged.
2. Rising Interest Rates and Shifting Investor Sentiment
The macroeconomic context in which LLM companies operate has changed significantly. Following years of historically low interest rates and abundant liquidity, central banks have adopted tighter monetary policies in response to inflationary pressures. As a result, the cost of capital has increased, and investor risk tolerance has diminished.
In this environment, high-burn-rate companies—particularly those without clear or proven monetization pathways—are encountering resistance. Venture capital firms are demanding more rigorous financial discipline, focusing on unit economics, path to profitability, and customer retention metrics rather than simply model performance or usage statistics. While AI remains a top priority for many funds, capital is no longer flowing indiscriminately. Startups that were once able to raise $100 million on a slide deck must now defend every dollar with credible business plans and growth trajectories.
3. Strategic Funding and Platform Dependence
An additional complication is the increasing dependence on strategic investors, particularly major cloud providers. Microsoft’s multi-billion-dollar investment in OpenAI, Google’s support of Anthropic, and Amazon’s backing of Anthropic and Hugging Face illustrate how the hyperscalers have positioned themselves at the center of the LLM funding ecosystem.
While these relationships provide essential compute resources and distribution channels, they also create asymmetrical power dynamics. Model providers often become deeply integrated into a specific cloud platform, reducing their negotiating leverage and long-term independence. Strategic investors may prioritize ecosystem alignment over standalone profitability, which can distort decision-making and complicate fundraising from neutral parties. Moreover, reliance on a single platform can alienate potential enterprise customers who are wary of vendor lock-in or who operate in multi-cloud environments.
4. High Burn Rates and Fragile Financial Models
The sheer cost of operating at the frontier of AI exacerbates financial fragility. As discussed in previous sections, training and deploying large models incurs substantial recurring costs. For many LLM companies, monthly burn rates exceed $20–50 million, driven by compute contracts, research staff, legal overhead, and enterprise support.
In the absence of robust and growing revenue streams, these expenses quickly deplete capital reserves. Even companies with large funding rounds are finding themselves returning to the fundraising table within 12 to 18 months, often at valuations that no longer support the original hype. This situation creates valuation compression risk—a phenomenon where subsequent rounds occur at flat or down valuations, which in turn erodes employee equity value and investor confidence.
Some companies attempt to compensate by raising even larger rounds from strategic backers, but this can delay rather than resolve the fundamental issue: unsustainable economics. Without a clear plan to generate operating profits—or at least achieve significant gross margins—capital alone cannot ensure long-term survival.
5. The IPO Path and Its Limitations
Given the capital intensity of the space, many LLM providers have eyed public markets as a potential exit or funding strategy. An initial public offering (IPO) offers access to a broader investor base, liquidity for early stakeholders, and greater brand legitimacy. However, the path to a successful IPO is fraught with challenges in the current environment.
Public market investors demand predictable financials, demonstrated customer demand, and scalability. Most LLM providers are still in the experimental or growth phases, with revenue figures that fall short of the thresholds typically required for public listing. Furthermore, the volatility associated with AI hype cycles makes valuations difficult to justify and maintain in the public arena.
There is also reputational risk. A poorly received IPO—due to weak earnings, governance concerns, or unclear business models—can damage a company’s long-term prospects and depress its stock for years. For these reasons, many AI firms are deferring IPO plans or exploring alternative liquidity events such as secondary share sales, mergers, or strategic acquisitions.
6. Funding Gaps for Second- and Third-Tier Players
While top-tier model providers may still command interest from investors, smaller or less differentiated companies are facing acute funding gaps. Many mid-stage startups that launched promising open-weight models or agent frameworks now find themselves squeezed between escalating costs and tepid revenue growth. Investor patience is wearing thin for companies that lack proprietary technology, enterprise traction, or unique go-to-market strategies.
This dynamic is likely to catalyze consolidation within the LLM ecosystem. We can expect a wave of mergers and acquisitions (M&A), where undercapitalized firms are absorbed by larger players seeking to bolster their talent pools, IP portfolios, or customer bases. While consolidation may create more resilient entities in the long run, it also signals a maturation of the market and a correction of earlier funding exuberance.
In sum, the funding landscape for large language model providers is undergoing a decisive shift. The once-abundant capital that fueled experimentation and hypergrowth is now constrained by economic realism and investor scrutiny. Companies must adapt by demonstrating operational discipline, refining monetization strategies, and preparing for more conservative capital markets. Those that cannot will likely fall victim to a new phase of consolidation, in which only the most efficient, differentiated, and strategically aligned players will endure.
Enterprise Adoption—High Hopes, Slow Uptake
From the outset of the generative AI boom, enterprises were viewed as the ultimate market frontier for large language model (LLM) providers. Armed with data-rich environments, complex workflows, and a perpetual need for productivity enhancement, large organizations seemed ideally positioned to benefit from LLMs. Providers projected that widespread enterprise adoption would drive high-margin, recurring revenues and enable sustainable business models. However, while interest remains strong, the pace and depth of enterprise LLM adoption have proven far more measured than originally anticipated. As a result, the expected revenue surge from the enterprise sector has yet to materialize at the scale many providers forecasted.
1. The Initial Surge in Interest
Following the release of ChatGPT and similar models, enterprises across industries began exploratory pilots to evaluate the applicability of generative AI. Use cases such as document summarization, legal contract analysis, code generation, customer service automation, and market intelligence were rapidly prototyped. Vendor partnerships were announced at a brisk pace, and internal innovation teams were tasked with integrating LLMs into existing platforms.
This initial wave of experimentation reflected genuine enthusiasm and a desire to capture first-mover advantages. LLM providers were quick to capitalize on the moment, launching enterprise-grade APIs, managed services, and cloud-based collaboration tools tailored for business users. Venture capital firms further amplified this momentum, emphasizing LLM-as-a-service (LLMaaS) as a compelling business-to-business (B2B) model.
Yet, as pilot programs transitioned to evaluations of full-scale deployment, enterprise decision-makers began to confront a complex reality. Issues related to cost, compliance, data governance, security, and integration surfaced, slowing the transition from testing to adoption.
2. Cost Justification Remains a Major Barrier
One of the most significant obstacles to broader enterprise LLM deployment is the difficulty in justifying return on investment (ROI). Unlike traditional software, where value delivery is often tightly coupled to specific business metrics, the benefits of LLMs can be diffuse and difficult to quantify.
For example, an AI assistant that drafts emails or summarizes meetings may increase employee efficiency but rarely in a way that translates directly into revenue or cost savings. In more technical domains, such as code generation or legal analysis, quality assurance processes still require substantial human oversight, which offsets some of the productivity gains.
These challenges are further compounded by the high cost of running LLMs at scale, particularly for real-time or high-volume use cases. Inference remains computationally expensive, and organizations that seek to leverage LLMs across thousands of users must factor in potentially millions of dollars in recurring infrastructure and subscription costs. Consequently, many enterprises have opted to limit usage to niche applications or internal proofs-of-concept.
3. Integration Complexities and Legacy Systems
Another barrier to LLM adoption is the difficulty of integrating models into legacy enterprise systems. Many organizations operate on deeply entrenched technology stacks with custom workflows, proprietary databases, and strict security protocols. LLM providers, however, often offer solutions that are cloud-native, externally hosted, and require extensive data movement to function effectively.
This technical mismatch necessitates significant engineering resources on the client side. It also raises concerns about data leakage, regulatory compliance, and vendor lock-in. For industries such as finance, healthcare, and government—where data sensitivity is paramount—these concerns can stall or completely block LLM adoption unless on-premises or fully isolated deployment options are provided.
While some vendors now offer fine-tuning and deployment capabilities within virtual private clouds (VPCs), these options often come at a premium and demand additional implementation effort. For many enterprises, the perceived risk-to-reward ratio remains unfavorable, especially in the absence of clear competitive advantage.
4. Organizational Readiness and Cultural Friction
Enterprise adoption is not solely a technical or financial matter—it is also cultural. Many organizations are still in the early stages of digital transformation and lack the internal expertise required to effectively deploy and manage LLM-powered systems. Concerns about job displacement, model errors, and ethical implications have sparked internal resistance among stakeholders, particularly in compliance-heavy departments.
Moreover, LLMs are probabilistic systems, meaning they do not guarantee deterministic outputs. This introduces unpredictability into enterprise workflows, which can be unacceptable in domains requiring high precision or auditability. Without robust guardrails, hallucinations, bias, and context misinterpretation remain persistent risks, further eroding executive confidence in full-scale deployment.
As a result, many enterprises have adopted a wait-and-see approach, monitoring technological developments while proceeding cautiously with integration efforts.
- Adoption Curve vs. Revenue Growth Projection
To illustrate the divergence between interest and monetization, a chart comparing enterprise adoption intent versus actual revenue growth from enterprise LLM offerings provides a useful perspective.
[Chart Placeholder: “Adoption Curve vs. Revenue Growth Projection for Enterprise LLM Offerings”]
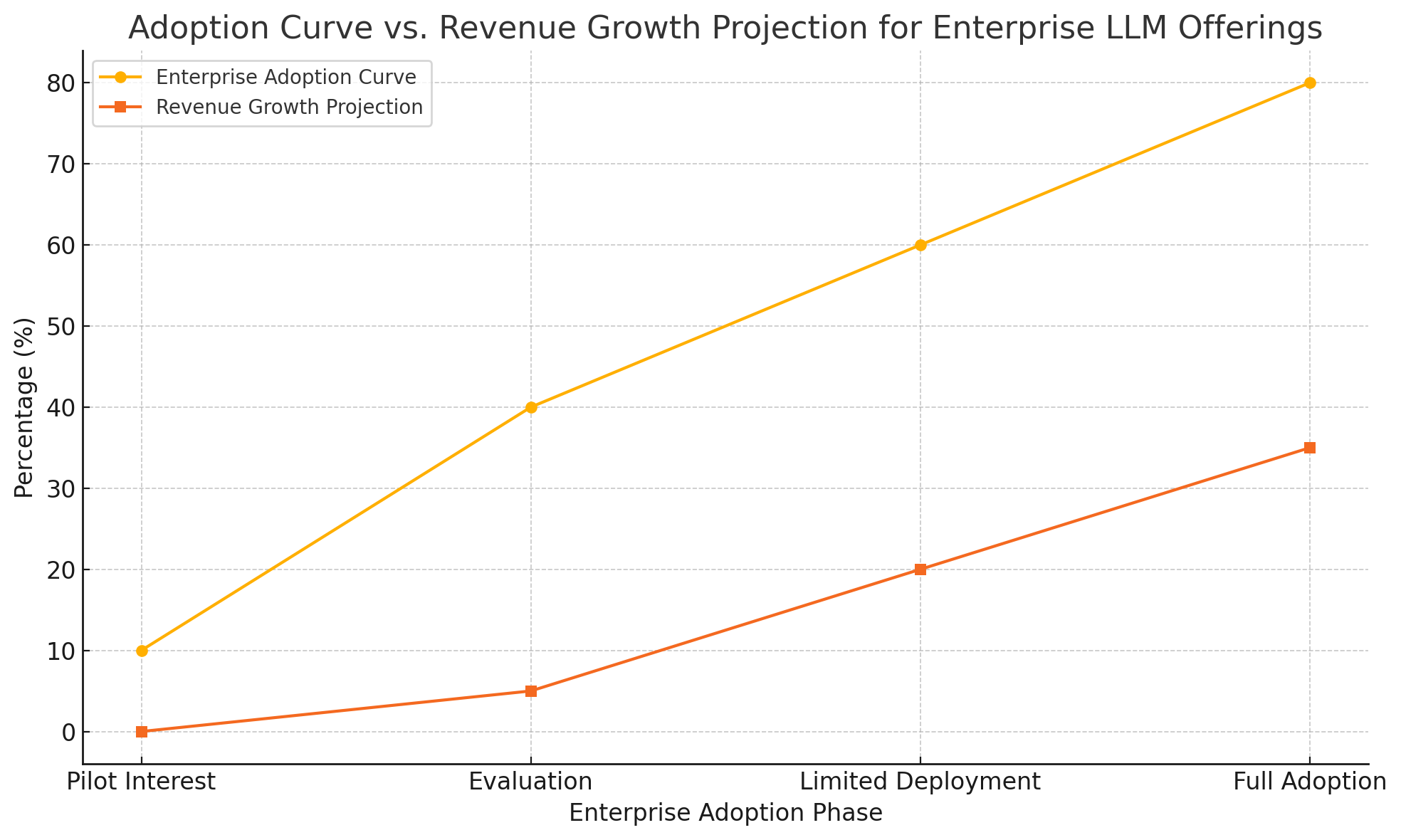
- The adoption curve shows rapid early experimentation and pilot development but slows significantly during procurement and integration phases.
- The revenue growth line reflects a lagging monetization effect, as only a small percentage of pilots convert into full-scale, paying deployments.
This gap highlights a central challenge: interest in LLMs does not immediately equate to scalable revenue, particularly in complex enterprise environments.
6. Customization Demands and the Role of Open Source
Enterprises increasingly demand customizable and controllable LLM solutions. This includes the ability to fine-tune models on proprietary data, control where and how models are hosted, and integrate seamlessly with internal APIs and knowledge bases.
Such preferences are driving interest in open-source models, which allow for on-premises deployment, modification, and lower total cost of ownership (TCO). Providers like Meta (LLaMA), Mistral, and Falcon have released performant open-weight models that enterprises can adapt without licensing fees or proprietary constraints.
This trend introduces competitive pressure for proprietary providers. Unless they can offer differentiated capabilities—such as superior performance, security assurances, or enterprise-specific tooling—they risk losing market share to self-managed alternatives that are perceived as more flexible and cost-efficient.
In summary, while enterprise demand for generative AI remains high, its translation into meaningful and sustained revenue for LLM providers has been slower than anticipated. A confluence of cost concerns, integration complexity, cultural inertia, and trust barriers continues to inhibit rapid deployment. Providers must address these challenges proactively by offering flexible deployment options, transparent pricing, robust safety features, and tailored enterprise support. Only then can the promise of generative AI in the enterprise context be fully realized—and monetized.
The Road Ahead—Consolidation, Regulation, and Strategic Pivots
As the initial exuberance around large language models (LLMs) gives way to a more measured understanding of their capabilities and limitations, providers are entering a pivotal phase. The financial pressures outlined in preceding sections—ranging from high infrastructure costs to stalled enterprise monetization—are compelling LLM companies to rethink their strategic orientation. What emerges is a landscape characterized by imminent consolidation, increasing regulatory scrutiny, and a necessary pivot toward more differentiated and sustainable business models.
1. Industry Consolidation: A Likely Recalibration
A period of market rationalization is all but inevitable. Over the past two years, a large number of companies entered the generative AI space, backed by substantial venture capital and armed with ambitious roadmaps. However, only a few have achieved significant revenue traction or brand differentiation. As the costs of sustaining operations mount and capital becomes more selective, the industry is poised for consolidation through mergers, acquisitions, and strategic partnerships.
The logic behind this trend is twofold. First, smaller or underfunded LLM providers may lack the resources to keep pace with advancements in model architecture, hardware optimization, and enterprise integration. Second, larger entities—including cloud hyperscalers, consulting firms, and vertical software providers—may view acquisition as a means to rapidly acquire talent, intellectual property, and market share.
This consolidation may mirror patterns observed in previous technological revolutions, such as cloud computing or enterprise SaaS, where a few dominant players eventually absorbed a multitude of early-stage entrants. In the context of LLMs, this would likely result in an ecosystem where a small number of foundational model providers are complemented by a diverse set of vertical or application-specific platforms.
2. The Rising Cost of Compliance and Regulation
As LLMs become more embedded in societal and economic systems, governments are accelerating regulatory frameworks aimed at ensuring transparency, accountability, and fairness in AI deployments. The European Union’s AI Act, the United States’ proposed executive orders, and similar efforts in China and Canada underscore the global momentum toward regulatory oversight.
For LLM providers, this shift introduces new financial and operational burdens. Compliance will require providers to implement robust documentation practices, publish transparency reports, conduct model risk assessments, and enable user-level controls for data usage and output auditing. These tasks are particularly complex for foundation models trained on vast and heterogeneous data sources, where lineage and attribution are often difficult to establish.
Moreover, legal exposure related to content generation—ranging from intellectual property infringement to misinformation dissemination—introduces liability risks that may necessitate expanded legal teams, insurance coverage, and content moderation infrastructure. These additional layers of governance, while necessary for public trust, further compound the cost of doing business and may disproportionately affect smaller providers with limited compliance capacity.
3. The Open-Source Threat and Hybrid Deployment Models
The surge of open-source LLMs presents both a challenge and an opportunity for commercial providers. Open-weight models such as Meta’s LLaMA, Mistral’s Mixtral, and Falcon have achieved performance benchmarks previously reserved for proprietary models. These models are increasingly adopted by developers and enterprises seeking cost-effective, customizable, and private deployments.
For commercial LLM providers, the growing popularity of open-source alternatives places downward pressure on pricing and shifts market expectations. In response, some companies are adopting hybrid models, where they offer both proprietary hosted solutions and open-source variants with optional enterprise support. This mirrors strategies employed in other software categories, such as databases and developer tools, where monetization occurs through premium features, service-level agreements (SLAs), and managed hosting.
This hybrid approach allows providers to maintain visibility and mindshare among developers while creating pathways to revenue in regulated or high-stakes environments. However, it also requires a delicate balance between openness and monetization, particularly in light of increasing competition and the potential for community-driven innovation to outpace proprietary roadmaps.
4. Strategic Pivots: From Generalization to Specialization
The prevailing strategy of training ever-larger general-purpose models is increasingly viewed as unsustainable. The escalating costs of compute, data, and model safety exceed the marginal gains achieved through scale alone. Consequently, many LLM providers are now pivoting toward specialization as a means to improve cost-efficiency and deliver more targeted value.
Specialization may take several forms:
- Domain-specific models trained on vertical data (e.g., legal, medical, financial) that outperform general-purpose models in specialized tasks.
- Use-case optimized models fine-tuned for specific applications such as contract analysis, customer service automation, or software development.
- Agentic frameworks that combine LLMs with tools, memory systems, and retrieval capabilities to execute multi-step tasks with higher reliability.
These pivots allow providers to move up the value chain and align their offerings more closely with business outcomes. Rather than competing purely on model size or benchmark scores, success in this new era will hinge on the ability to solve real problems in constrained environments with measurable efficiency.
5. Business Model Innovation: Beyond API Monetization
The limitations of traditional API monetization—discussed earlier in the blog—are prompting exploration of alternative revenue models. Among the emerging strategies are:
- AI-as-a-Service (AIaaS) platforms that bundle infrastructure, models, and application layers into turnkey solutions.
- Outcome-based pricing, where customers pay based on business results such as cost savings, lead generation, or reduced customer churn.
- White-label offerings that allow enterprises to brand and integrate LLMs into their own products without attribution.
- AI marketplaces that enable third-party developers to build and commercialize plugins, agents, or workflows atop a foundational LLM layer.
These models aim to create more defensible revenue streams while expanding the addressable market. However, they also demand increased operational complexity, including billing integration, customer success management, and support infrastructure.
6. Outlook: Strategic Differentiation as a Survival Imperative
The future of the LLM industry will be shaped not solely by technical prowess but by strategic positioning and execution. Providers that succeed will be those that combine technological innovation with clear product-market fit, robust regulatory compliance, and financial discipline. They will need to differentiate through vertical focus, reliability, enterprise readiness, and ecosystem development—not simply through model scale.
Consolidation will reward providers with strong balance sheets and clear go-to-market strategies. Regulation will favor those that embrace transparency and governance. And hybrid approaches that combine open models with enterprise-grade tooling may offer a middle path between commoditization and control.
In conclusion, the road ahead for large language model providers is marked by both existential risks and transformative opportunities. The era of unchecked spending and speculative growth is drawing to a close. In its place emerges a more disciplined and competitive environment where only those who can adapt—strategically, financially, and operationally—will thrive. The next chapter of generative AI will not be written solely in code or research papers, but in boardrooms, compliance offices, and customer deployments. The stakes are high, and the margin for error is narrowing.
Conclusion
The transformative promise of large language models (LLMs) has captivated industries, policymakers, and technologists alike. These systems have demonstrated unprecedented capabilities in language generation, comprehension, and task automation—laying the foundation for a new era of human-computer interaction. However, as the sector matures, the economic and operational realities underpinning LLM development are becoming impossible to ignore. Beneath the surface of exponential innovation lies a complex financial architecture that poses existential risks to the sustainability of many LLM providers.
Throughout this analysis, we have examined the multifaceted financial pressures confronting companies that develop and deploy large-scale language models. From the significant capital expenditures required for model training and infrastructure to the ongoing operational costs associated with inference, monitoring, and compliance, the financial burden is immense. These pressures are exacerbated by monetization models that, while promising in theory, often fail to deliver the unit economics necessary for long-term viability. API-based pricing, freemium conversions, and cloud-based integrations are proving insufficient—particularly when faced with growing competition from open-source alternatives and internal enterprise deployments.
The funding environment, once characterized by exuberance and rapid capitalization, is entering a more cautious phase. Investor sentiment has shifted from growth-at-all-costs to disciplined value creation, placing intense scrutiny on business models, revenue pipelines, and cost management. Strategic backers, while still active, increasingly seek alignment with broader ecosystem goals rather than supporting LLM providers as standalone ventures. As a result, many companies are discovering that high valuations and strong user engagement do not necessarily equate to financial resilience.
Enterprise adoption—long considered the primary monetization pathway—has proven slower and more fragmented than expected. While interest remains robust, full-scale deployment has been hindered by integration challenges, ROI ambiguity, and compliance concerns. Enterprises are demanding greater customization, control, and security—demands that increase implementation complexity and dilute near-term revenues. Moreover, open-source models continue to improve in performance and accessibility, enabling organizations to develop and deploy generative AI capabilities without relying on commercial APIs or hosted platforms.
In light of these pressures, the industry is undergoing a necessary transformation. Consolidation appears inevitable as undercapitalized players struggle to sustain operations, and more resource-rich incumbents seek to acquire talent, technology, and market share. Regulatory mandates are also reshaping the cost structure and operational frameworks required to compete in global markets. Increasingly, differentiation will depend not only on technical performance but also on the ability to deliver safe, transparent, and compliant systems.
Looking ahead, the most viable path forward involves strategic pivots and business model innovation. General-purpose models trained at ever-larger scales may no longer be economically tenable for all but the most well-funded entities. Instead, specialization—whether by industry, use case, or application type—offers a more efficient and focused route to value creation. Additionally, providers must experiment with monetization models that align more closely with customer outcomes, such as enterprise subscriptions, white-label solutions, and AI-enabled workflow automation.
Crucially, the coming phase of the LLM ecosystem will be defined not just by technological capability but by the ability to balance innovation with financial discipline. The providers that endure will be those who successfully navigate this equilibrium—adapting to market signals, meeting enterprise needs, and complying with evolving regulations, all while continuing to invest in the underlying science of language modeling.
In conclusion, while the promise of LLMs remains vast, their future will hinge on more than just performance benchmarks and research breakthroughs. Financial sustainability, strategic clarity, and operational adaptability will determine which providers lead the next wave of generative AI and which are left behind. The industry stands at a critical juncture, and the decisions made now will shape not only the competitive landscape, but the trajectory of artificial intelligence itself in the decades to come.
References
- OpenAI Pricing Overview
https://openai.com/pricing - Anthropic Claude API Documentation
https://docs.anthropic.com/claude - Microsoft and OpenAI Partnership
https://blogs.microsoft.com/blog/2023/01/23/microsoft-announces-third-phase-of-long-term-partnership-with-openai/ - Google's Gemini AI Introduction
https://blog.google/technology/ai/google-gemini-ai/ - Meta AI’s Open Source Models (LLaMA)
https://ai.meta.com/llama/ - Mistral AI Model Releases
https://mistral.ai/news/announcing-mistral-7b/ - Hugging Face Model Hub
https://huggingface.co/models - Stability AI’s Open LLMs
https://stability.ai/news/stability-ai-launches-stablelm-suite-of-language-models - EU Artificial Intelligence Act Overview
https://digital-strategy.ec.europa.eu/en/policies/european-approach-artificial-intelligence - U.S. Executive Order on Safe, Secure, and Trustworthy AI
https://www.whitehouse.gov/briefing-room/statements-releases/2023/10/30/fact-sheet-president-biden-issues-executive-order-on-safe-secure-and-trustworthy-artificial-intelligence/ - Gartner Report on Generative AI Enterprise Adoption
https://www.gartner.com/en/articles/generative-ai-will-transform-your-enterprise - NVIDIA’s Financial Disclosures on AI Demand
https://www.nvidia.com/en-us/about-nvidia/investor-relations/ - Databricks and MosaicML Merger Announcement
https://www.databricks.com/blog/2023/06/26/databricks-announces-agreement-to-acquire-mosaicml.html - Amazon and Anthropic Strategic Investment
https://www.aboutamazon.com/news/innovation-at-amazon/amazon-anthropic-strategic-collaboration - The AI Index Report by Stanford HAI
https://aiindex.stanford.edu/report/