Rise of the Robotic Consumer: How Autonomous Systems Are Reshaping Commerce Across Industries
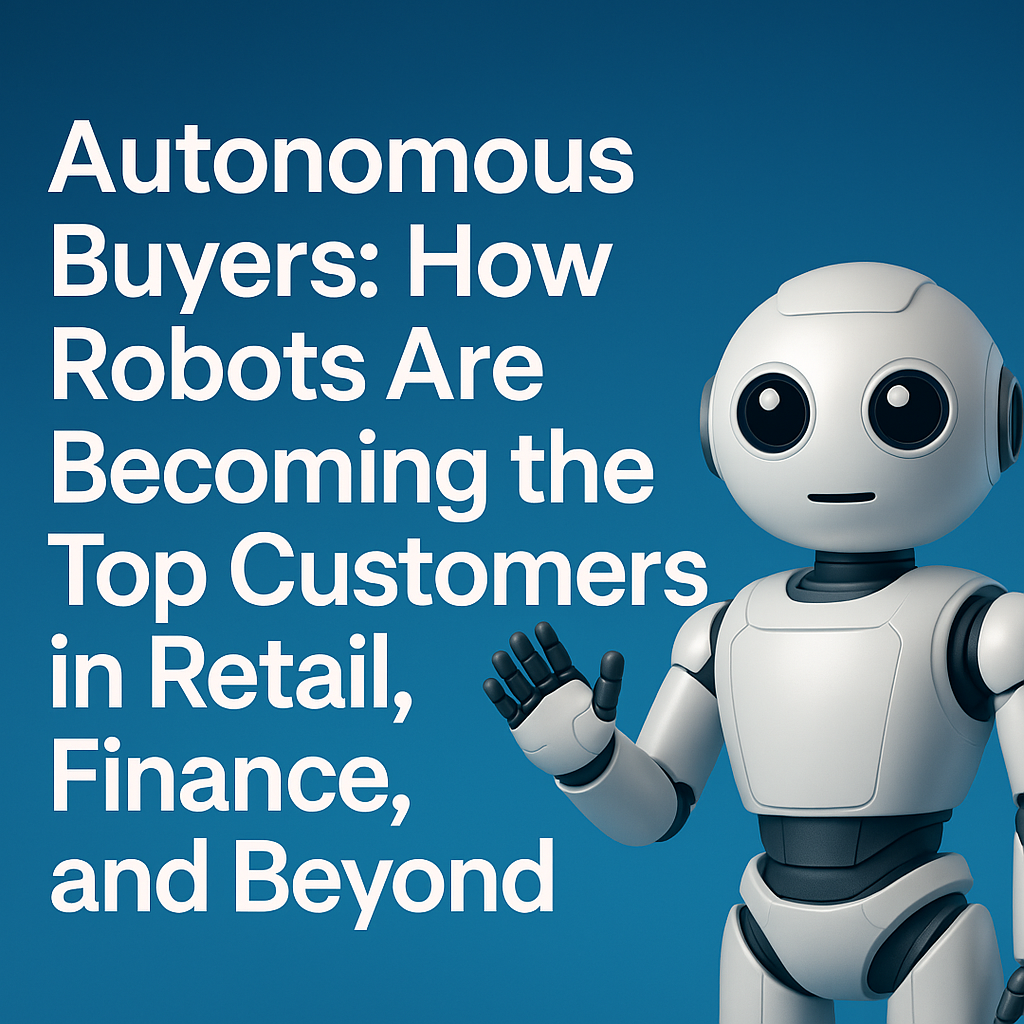
In the evolving landscape of commerce and industry, the role of robotics has traditionally been confined to the domains of manufacturing, logistics, and repetitive task automation. However, recent advancements in artificial intelligence, connectivity, and autonomous systems have given rise to a transformative trend: robots are no longer merely tools executing human commands—they are emerging as independent clients and economic actors. These robotic systems are increasingly capable of making purchasing decisions, managing supply chains, and engaging in transactions with minimal or no human intervention.
This paradigm shift is being fueled by rapid developments in machine learning, multi-agent coordination, and programmable procurement interfaces. Autonomous robots—whether embedded in physical form, such as warehouse bots and delivery drones, or virtual agents, such as AI-driven avatars and digital assistants—are now operating as repeat customers in retail environments, procurement platforms, and service-oriented ecosystems. The result is a profound reconfiguration of customer identity and market dynamics.
The implications of this shift are far-reaching. For retailers, robots represent a new category of high-frequency, precision-driven clients who demand seamless, API-compatible experiences and real-time inventory fulfillment. For suppliers, the rise of robotic clients necessitates adaptations in product lifecycle planning, forecasting models, and service agreements. And for the broader economy, the inclusion of autonomous systems as consumers introduces questions about accountability, legal rights, and the architecture of machine-to-machine commerce.
This blog post explores the expanding role of robots as top clients in retail and beyond. It examines the technological drivers behind this trend, showcases its early applications, assesses the implications for traditional business models, and forecasts the opportunities and challenges that lie ahead. Through this lens, we aim to understand how the consumer of the future may, increasingly, not be a human—but a machine acting on behalf of one, or entirely on its own.
The Rise of Robots as Autonomous Buyers
The concept of robots acting as independent consumers may have once seemed relegated to the realms of speculative fiction, yet it is rapidly becoming a tangible reality in modern economic ecosystems. The transformation from programmed tool to autonomous buyer is a significant technological milestone that reflects advances in artificial intelligence (AI), machine learning (ML), edge computing, and sensor integration. These developments are enabling robotic systems not only to perform operational tasks but also to initiate, execute, and optimize purchasing decisions—a function traditionally reserved for human actors.
From Passive Tools to Intelligent Agents
The early generation of robots functioned exclusively under the direction of human operators, primarily within controlled industrial settings. Their capabilities were confined to routine, repetitive tasks such as welding, assembly, or palletizing. However, with the maturation of embedded AI and the incorporation of real-time data processing, today’s robotic systems exhibit a level of situational awareness and decision-making autonomy that is fundamentally different from their predecessors.
This evolution is particularly evident in robotics platforms that combine hardware mobility with sophisticated software decision layers. These machines are now able to recognize supply needs, identify qualified vendors, initiate procurement requests, and even track delivery schedules—all without human oversight. For example, modern warehouse robots can detect when spare parts or consumables are running low, trigger restocking procedures through integrated enterprise resource planning (ERP) systems, and manage their own maintenance cycles through intelligent diagnostics.
The use of autonomous decision-making frameworks, such as reinforcement learning and policy optimization algorithms, allows these systems to learn from past transactions, evaluate supplier performance, and adapt procurement strategies based on historical efficiency. Such capabilities are instrumental in reducing downtime, optimizing inventory, and ensuring business continuity in highly dynamic environments.
Architectures Enabling Autonomous Purchasing
At the heart of this transformation lies a convergence of several enabling technologies. Multi-agent systems (MAS), for instance, allow networks of robots to communicate and collaborate in executing distributed tasks, including joint purchasing strategies. This is particularly useful in shared logistics hubs, where robots coordinate procurement to reduce redundancy and take advantage of bulk ordering discounts.
Simultaneously, large language models (LLMs) and natural language processing (NLP) frameworks have been integrated into robotic systems, allowing them to parse vendor catalogs, interpret product specifications, and interface with customer support systems via APIs or chat interfaces. These LLM-powered agents can act as purchasing managers, negotiating contract terms, comparing offers, and managing digital workflows with a level of nuance previously thought exclusive to human intelligence.
Furthermore, blockchain technology and smart contracts are emerging as critical components in the evolution of autonomous procurement. These tools offer a secure and transparent infrastructure for robotic systems to execute conditional transactions with vendors, ensuring trust, compliance, and traceability. This capability is especially useful in industries where regulatory standards must be rigorously enforced, such as pharmaceuticals or aerospace manufacturing.
Notable Use Cases and Real-World Deployments
Several real-world examples illustrate the practical adoption of robots as autonomous buyers across different industries. In the e-commerce and logistics sectors, companies such as Amazon and Alibaba have deployed fleets of intelligent warehouse robots capable of initiating purchase orders for inventory replenishment. These robots assess stock levels, analyze sales velocity, and coordinate with backend procurement systems to ensure optimal product availability.
In the automotive manufacturing industry, robotic arms equipped with embedded AI are capable of monitoring tool wear and triggering the purchase of replacement components before failure occurs. This predictive maintenance purchasing not only reduces downtime but also contributes to overall operational efficiency.
In agriculture, autonomous tractors and irrigation bots have begun to purchase seeds, nutrients, and pesticide supplies based on soil data and weather forecasts. These purchases are determined through AI models trained on years of crop yield data, satellite imagery, and regional market prices. The result is a form of precision agriculture, where machines make economically and ecologically optimized decisions.
Meanwhile, in the smart home and consumer electronics domain, virtual assistants such as Amazon Alexa and Google Assistant are inching toward becoming autonomous retail agents. These devices can now reorder household goods based on consumption patterns, user preferences, and delivery constraints, offering a glimpse into how domestic robots may one day dominate routine consumer purchasing.
Economic and Strategic Implications
The emergence of robots as autonomous clients introduces profound economic implications. First, it redefines the traditional concept of a customer, expanding it to include algorithmic and mechanical actors with consistent, predictable, and data-driven purchasing behavior. This evolution challenges existing customer relationship management (CRM) frameworks and compels businesses to rethink loyalty, engagement, and retention strategies for non-human clients.
Second, robotic buyers typically operate with fewer cognitive biases and more rational heuristics compared to their human counterparts. Their decision-making is based on empirical data, statistical inference, and performance history. This could lead to a shift in marketing strategies, where emotional appeal and brand storytelling give way to algorithmic optimization and feature-value targeting.
Third, the economic scale of robotic clients could outstrip that of traditional consumers. A single robotic system, operating 24/7, may initiate hundreds or thousands of transactions annually, especially in high-frequency domains such as manufacturing, retail restocking, or delivery logistics. This behavior positions robots as high-value, repeat customers, thereby altering the demand landscape and prompting vendors to develop specialized pricing, support, and fulfillment strategies for machine clients.
Challenges and Limitations
Despite the promise, the adoption of robots as buyers is not without challenges. One major concern lies in cybersecurity. Autonomous agents, if compromised, could cause significant financial loss or supply chain disruption through malicious purchases or denial-of-service attacks on procurement systems. Robust encryption, identity management, and transactional safeguards are essential to mitigating such risks.
Another issue is ethical accountability. When a robot makes a faulty or non-compliant purchase, determining liability becomes complex. Does the fault lie with the manufacturer, the software provider, the network operator, or the AI itself? Legal frameworks are still in their infancy when it comes to defining robotic agency in commerce.
Lastly, there remains the challenge of standardization and interoperability. For autonomous robots to effectively engage in commerce across different vendors, platforms, and jurisdictions, there must be common protocols, data formats, and transaction standards. Organizations such as the IEEE and ISO are beginning to address these gaps, but widespread implementation remains a work in progress.
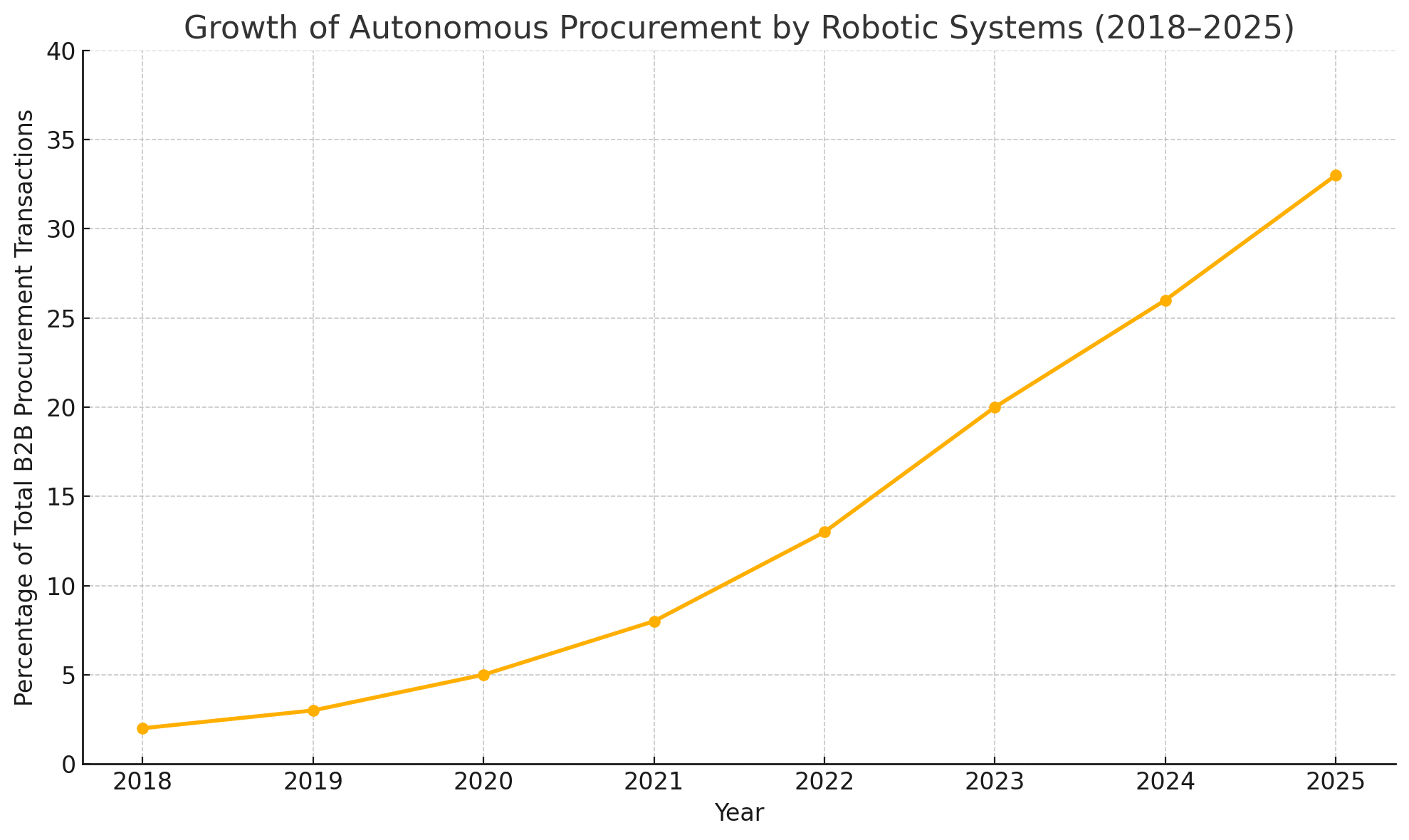
In summary, the rise of robots as autonomous buyers marks a turning point in the automation narrative. It signals a transition from passive execution to active economic participation, driven by technological sophistication, operational necessity, and the increasing complexity of modern commerce. As robotic systems gain more autonomy, businesses must evolve accordingly—adapting their platforms, processes, and policies to cater to this new class of highly efficient, data-driven, and scalable clients.
Retail Transformation: Robots as Repeat Buyers
The retail industry, historically shaped by the preferences and behaviors of human consumers, is undergoing a profound transformation. As robots and autonomous systems evolve into consistent, decision-making entities, they are beginning to emerge as repeat buyers in retail ecosystems. These robotic clients, empowered by artificial intelligence, operate on behalf of organizations or even other machines, purchasing products, replenishing inventories, and optimizing procurement cycles without direct human involvement. This evolution marks a significant shift in the retail paradigm—one that demands new technologies, business strategies, and engagement models.
Autonomous Agents as Retail Customers
In traditional retail models, customer behavior has been characterized by variability, emotion, and unpredictability. Human consumers make decisions based on convenience, brand loyalty, price sensitivity, and subjective preferences. Robotic buyers, in contrast, operate with consistency, precision, and algorithmic discipline. They make purchasing decisions based on predefined criteria such as availability, unit cost, delivery times, historical performance, and compliance standards.
These robotic buyers are often integrated into broader enterprise systems, functioning as procurement modules that autonomously trigger purchases when inventory thresholds are reached. Unlike traditional just-in-time systems, which rely on human oversight, robotic purchasing agents continuously monitor stock levels, forecast usage based on real-time analytics, and interface with vendors through application programming interfaces (APIs). The result is a self-sustaining supply loop, where machines autonomously maintain optimal inventory levels.
For instance, an autonomous retail restocking bot deployed in a smart convenience store can evaluate product turnover using embedded sensors, cross-reference this data with consumer trends, and reorder items from suppliers as needed. These bots can make multiple micro-purchases throughout the day, creating a dynamic inventory management system that minimizes waste, reduces holding costs, and maximizes shelf availability.
API-Driven Commerce and Machine-to-Machine Marketplaces
Central to this transformation is the adoption of API-driven commerce, where digital storefronts and backend systems are designed for seamless integration with autonomous clients. Retailers are now developing machine-readable product catalogs, transactional APIs, and automated negotiation protocols that enable robotic clients to interact directly with their systems. These interfaces remove the need for human intervention in the purchasing process, creating a streamlined and scalable B2B2M (business-to-business-to-machine) commerce environment.
Machine-to-machine (M2M) marketplaces represent the next stage of this evolution. These digital ecosystems allow robotic systems from different organizations to interact, transact, and even engage in cooperative purchasing. For example, multiple delivery drones operated by different companies might jointly purchase bulk fuel supplies or maintenance services through a shared marketplace. This collaborative behavior is facilitated by autonomous negotiation protocols and smart contracts, which enable multi-agent procurement agreements based on shared goals or constraints.
Additionally, machine clients may participate in dynamic bidding environments, where they assess real-time market conditions and algorithmically select vendors based on price, reliability, or delivery speed. Retailers offering programmatic access to inventory and flexible pricing models are well-positioned to capitalize on this shift, as robotic clients inherently favor suppliers that offer automation-friendly platforms.
Impact on Loyalty, Pricing, and Customer Retention
Robotic clients represent a fundamentally different customer profile, and their behavior challenges long-held assumptions about brand loyalty and customer engagement. Unlike human consumers who may develop emotional attachments to brands, robotic systems are more likely to prioritize quantitative metrics such as cost-efficiency, performance consistency, and fulfillment accuracy. Consequently, the traditional tools of retail marketing—branding, storytelling, promotional campaigns—may have diminished relevance in influencing robotic purchasing behavior.
Instead, robotic clients tend to exhibit loyalty only insofar as a vendor consistently meets or exceeds algorithmic performance thresholds. If a supplier's API slows down, if delivery accuracy declines, or if pricing becomes non-competitive, robotic systems will automatically switch to a better-performing alternative. This creates an environment of perfect competition, where continuous optimization is required to retain robotic clients.
However, it is important to note that robotic buyers do not inherently lack loyalty—they simply express it differently. A vendor offering high service-level agreement (SLA) reliability, excellent integration tools, and historical fulfillment accuracy may earn long-term robotic repeat business, even in the absence of pricing advantages. In this way, customer retention becomes a function of technical performance rather than emotional appeal.
Retailers are beginning to respond to these shifts by offering robot-targeted loyalty programs, such as API call discounts for high-volume machine clients, preferred access to time-sensitive inventory, or predictive analytics tools that assist robotic buyers in optimizing their procurement strategies.
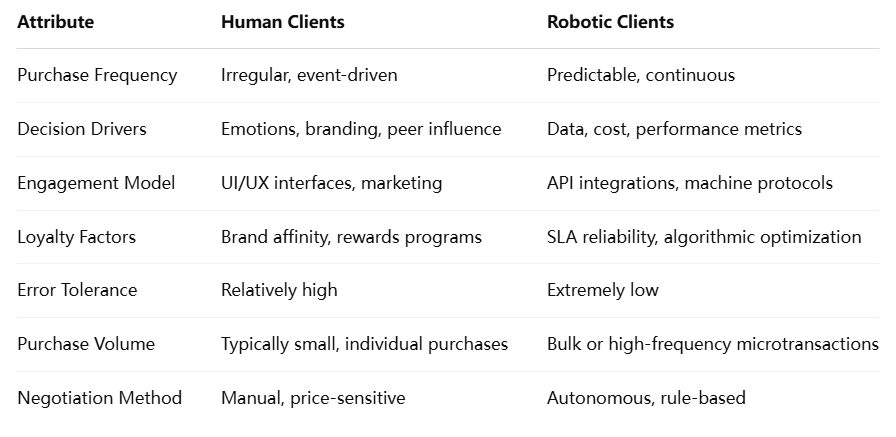
Case Studies and Retail Adaptation
Several prominent retailers and technology firms are leading the charge in adapting their platforms to accommodate robotic clients. Amazon, for instance, has developed an extensive suite of APIs that enable machine-to-machine interactions, allowing autonomous warehouse bots and third-party vendor systems to order, restock, and distribute products with minimal human oversight. The company’s fulfillment centers are optimized for both human and robotic purchasing cycles, balancing B2C and M2M transactions in real-time.
Similarly, JD.com in China has implemented a fully automated warehouse in Shanghai where autonomous systems manage inventory, trigger purchases, and replenish goods in a closed-loop system. The warehouse’s intelligent management software coordinates with upstream suppliers and logistics providers using M2M communications to ensure uninterrupted operations.
Retail technology firms such as Ocado, which specializes in automated grocery fulfillment, have built entire business models around serving both human and robotic clients. Their systems are designed to interface with autonomous bots that replenish household items based on consumption patterns and nutritional needs, using AI algorithms that predict dietary habits and recommend optimal purchase combinations.
These examples illustrate a broader trend in the industry: the emergence of robot-centric retail infrastructure. Retailers are not only building interfaces for robotic clients but also redesigning internal workflows, supply chains, and service architectures to support a new generation of non-human customers.
Strategic Implications for Retailers
The rise of robotic repeat buyers presents retailers with both significant opportunities and strategic challenges. On the one hand, robotic clients offer predictable demand, consistent purchasing cycles, and minimal customer service costs. On the other hand, they demand high levels of integration, real-time responsiveness, and unwavering reliability.
To succeed in this new landscape, retailers must prioritize:
- API-first architecture: Ensuring systems are accessible to autonomous clients via secure, scalable APIs.
- Real-time data synchronization: Offering up-to-date inventory, pricing, and shipping data for machine parsing.
- Adaptive fulfillment networks: Supporting low-latency, high-accuracy delivery for robotic supply chains.
- Performance-based loyalty models: Rewarding machine clients based on usage, uptime, and compliance.
Moreover, forward-thinking retailers may choose to develop dedicated robotic customer success teams—not composed of human account managers, but of monitoring and support systems designed to ensure seamless robotic engagement. These platforms would include diagnostic tools, anomaly detection modules, and automated resolution agents to address integration or transaction issues in real time.
In conclusion, the retail sector is at the forefront of a significant transformation, driven by the integration of autonomous robotic buyers. As these systems become more prevalent and more capable, they will redefine the dynamics of retail purchasing, loyalty, and fulfillment. Retailers that adapt to this shift by embracing machine-first commerce principles and investing in robotic customer infrastructure will be well-positioned to thrive in a marketplace where the most valuable clients may no longer be human—but highly intelligent, autonomous systems operating with speed, scale, and precision.
Impacts on Supply Chains and Vendor Strategy
The emergence of robots as autonomous clients is not merely a technological novelty—it represents a structural evolution with profound implications for supply chains and vendor strategies. As robotic systems assume the role of repeat buyers, their unique behavioral patterns and operational characteristics are compelling suppliers and logistics providers to reconfigure their models of service delivery, inventory management, and demand forecasting. These changes are ushering in a new era of precision logistics and machine-centric value chains that prioritize responsiveness, integration, and continuous optimization.
Predictability and Efficiency in Robotic Procurement
One of the most significant advantages of serving robotic clients is the predictability they offer. Unlike human customers, whose purchasing behaviors can be sporadic and influenced by emotional or seasonal factors, robotic buyers operate based on algorithmic logic and scheduled triggers. Their decisions are informed by real-time consumption data, forecast models, and predefined thresholds, allowing vendors to anticipate orders with a high degree of accuracy.
This predictability enables just-in-time (JIT) and just-in-case (JIC) inventory strategies to coexist more effectively. While JIT systems minimize holding costs by aligning inventory levels closely with actual demand, JIC systems buffer against uncertainty. Robotic buyers help strike a balance between these approaches by delivering consistently accurate demand signals, thereby enabling suppliers to optimize inventory without overstocking or risking shortages.
Additionally, the frequency and scale of robotic transactions allow for more granular replenishment cycles. Rather than large batch orders placed intermittently, robotic clients tend to initiate smaller, more frequent purchases, particularly in high-turnover or high-precision sectors. This shift supports a more fluid supply chain model, reducing waste, improving cash flow, and enhancing inventory agility.
Transformation of Vendor-Customer Relationships
The traditional vendor-customer relationship, which relies heavily on human interaction, account management, and relationship-building, is being fundamentally altered. In the context of robotic clients, performance replaces persuasion as the primary mechanism of retention. Vendors are judged by their ability to deliver speed, accuracy, uptime, and seamless integration—not by branding, personal rapport, or customer service.
This transformation compels vendors to invest in digital service layers, including robust APIs, transactional logging, uptime guarantees, and real-time error resolution protocols. These capabilities are essential to maintaining trust and reliability with robotic systems, which evaluate suppliers continuously based on objective performance metrics.
Moreover, the procurement process is becoming increasingly data-driven and automated. Vendor selection algorithms employed by robotic clients typically weigh factors such as historical delivery performance, order accuracy, unit pricing, and latency. This requires vendors to maintain high-quality metadata, up-to-date pricing APIs, and transparent service-level data to remain competitive in autonomous marketplaces.
In some cases, robotic clients are empowered to switch vendors dynamically in response to performance deviations. This means that a single late shipment or API outage could lead to immediate disqualification from the robotic client's approved vendor list. The margin for error is extremely narrow, and the emphasis on continuous optimization is paramount.
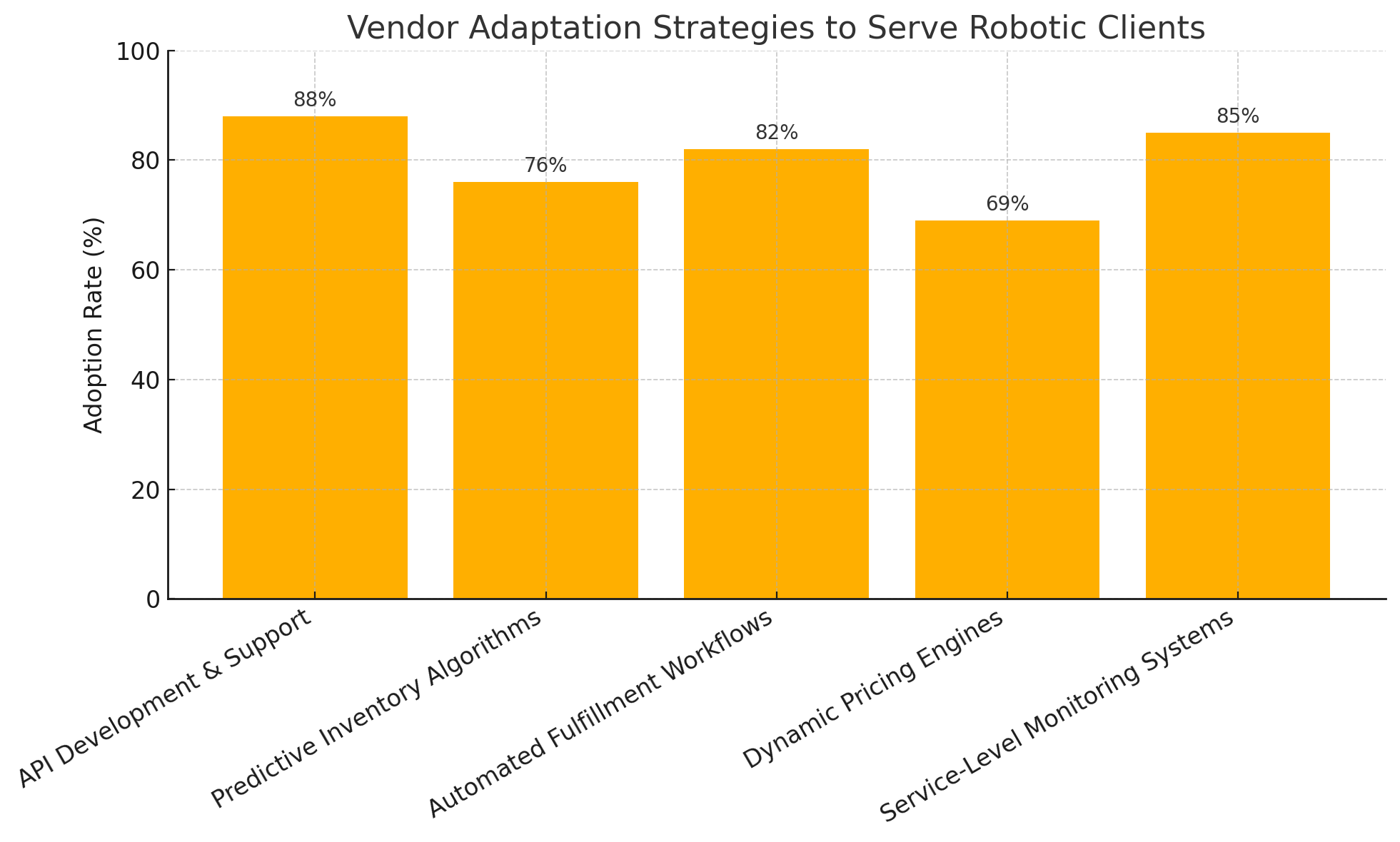
The Rise of Machine-Optimized SLAs
Service-level agreements (SLAs) are evolving to accommodate the expectations of robotic clients. These clients require machine-optimized SLAs that define thresholds for latency, response time, delivery accuracy, and integration reliability. Traditional SLAs, which are often structured around human accountability and manual resolution pathways, are being replaced by automated monitoring and enforcement mechanisms that respond in real time.
For example, a robotic client placing orders through an API may expect a response time under 100 milliseconds, fulfillment within a 12-hour window, and delivery confirmation via a machine-readable JSON protocol. Any deviation from these metrics can trigger automated escalation, rerouting to alternative vendors, or the invocation of smart contract penalties.
Vendors must therefore deploy automated SLA monitoring dashboards capable of interfacing directly with robotic systems. These tools track performance in real time, generate compliance reports, and trigger corrective actions without requiring human intervention. In effect, vendor accountability is increasingly being adjudicated by machines, and performance transparency becomes a competitive differentiator.
Furthermore, machine-optimized SLAs can include predictive clauses, where vendors commit to proactive stock management based on robotic client usage patterns. This collaborative forecasting model strengthens vendor-client relationships and reduces friction in the supply chain.
Integration with ERP and Supply Chain Systems
To serve robotic clients effectively, vendors must ensure seamless integration between their enterprise resource planning (ERP) systems and robotic procurement platforms. This integration enables end-to-end automation across procurement, production, warehousing, and distribution functions.
Key to this integration is the development of event-driven architectures that can respond dynamically to real-time events generated by robotic clients. For instance, when a factory robot detects a component failure and initiates a purchase order, the vendor’s ERP system must validate inventory, trigger picking operations, schedule dispatch, and update the robotic client—all within minutes. This level of orchestration requires cloud-native infrastructure, microservices, and asynchronous processing pipelines.
Moreover, the integration between ERP systems and robotic clients supports closed-loop supply chain analytics. Vendors can gain insights into client behavior, consumption patterns, and usage anomalies, which can inform predictive maintenance offerings, upsell opportunities, and co-development of specialized products for machine clients.
This data-rich environment enables a more collaborative vendor-client relationship, where feedback loops are accelerated and innovation cycles are compressed. Vendors that leverage robotic client data to iterate on product features, packaging, or logistics models can achieve faster time-to-market and higher client satisfaction.
Challenges in Vendor Adaptation
While the potential benefits of serving robotic clients are substantial, vendors face several challenges in adapting to this new paradigm. The foremost among these is technological readiness. Not all suppliers possess the infrastructure or technical expertise to expose real-time APIs, automate fulfillment workflows, or manage autonomous SLAs. Small and mid-sized enterprises (SMEs), in particular, may struggle to meet the integration standards required by robotic clients.
Cybersecurity is another major concern. As robotic clients engage in high-frequency, high-value transactions, they become attractive targets for cyber threats. Vendors must implement end-to-end encryption, secure authentication, and anomaly detection mechanisms to safeguard transactional integrity and protect client systems from malicious interference.
Legal and regulatory ambiguity also presents complications. Contracts executed between robots and vendors may raise questions about enforceability, liability, and jurisdiction. In the absence of standardized frameworks governing machine-to-machine commerce, vendors must proceed with caution, particularly in regulated industries.
Finally, there is the challenge of human workforce transition. As robotic clients demand fully automated interfaces, the role of traditional sales and account management teams may diminish. Vendors must retrain personnel, redefine roles, and develop new competencies in API management, robotic customer support, and machine learning-driven business analysis.
In conclusion, the integration of robots as repeat buyers into retail supply chains is reshaping how vendors approach service delivery, system architecture, and customer engagement. The rise of robotic clients demands a shift from relationship-based selling to performance-based contracting, from periodic fulfillment to real-time synchronization, and from manual oversight to automated accountability. Vendors that recognize and embrace these changes will not only maintain relevance but also unlock new avenues of efficiency, scale, and innovation in the rapidly evolving machine economy.
Expansion Beyond Retail: Robots as Clients in Other Sectors
While the retail industry has emerged as an early adopter of robot-to-business (R2B) transactions, the phenomenon of robots functioning as autonomous clients is gaining traction far beyond this domain. Across various sectors—ranging from finance and healthcare to agriculture and logistics—intelligent machines are beginning to act as independent economic agents. These robotic clients are executing purchases, managing service subscriptions, and initiating procurement workflows with a level of autonomy that fundamentally alters traditional business models.
The expansion of robotic clientele into these sectors signals a broader economic transformation wherein machines are not just tools of production or assistance but consumers of services, resources, and data in their own right. This evolution raises critical questions about system interoperability, accountability, governance, and the future of human-machine interaction in commercial ecosystems.
Finance: Robo-Advisors and Autonomous Capital Allocation
The financial services sector has already seen significant disruption through the rise of robo-advisors—automated platforms that manage investment portfolios using algorithmic strategies. Initially conceived to serve human clients, these platforms are increasingly being adapted to manage capital on behalf of robotic or AI-based entities.
For example, autonomous systems responsible for managing digital infrastructure may allocate budgetary resources toward computing power, cloud services, or API subscriptions based on performance metrics. These decisions are executed in real time, bypassing human oversight entirely. In high-frequency trading environments, algorithmic agents independently buy and sell financial instruments based on predictive modeling, market sentiment analysis, and programmed thresholds. These agents, though not embodied as physical robots, function as economic clients interacting with financial systems, executing complex contracts and reallocating capital without direct human instruction.
Furthermore, decentralized autonomous organizations (DAOs) in the blockchain space are emerging as collective robotic entities capable of deploying capital, voting on investment strategies, and engaging in contractual agreements—effectively acting as clients of traditional financial infrastructure and digital marketplaces.
Healthcare: Medical Robots as Procurement Agents
In healthcare, the increasing use of medical robotics has introduced new efficiencies in surgery, diagnostics, and patient care. However, a less visible but equally transformative trend is the ability of these systems to procure resources and services autonomously. Advanced surgical robots, for instance, can monitor tool usage and initiate reorders of surgical components when thresholds are reached. Diagnostic machines may automatically purchase reagents or maintenance services based on usage patterns and operational performance.
Hospital management platforms integrated with robotic systems can track supply utilization across departments and trigger automated procurement actions. This is particularly relevant in high-pressure environments where human error or delayed purchasing could compromise patient care. By leveraging robotic clients, healthcare providers gain a self-sustaining supply chain that improves reliability, reduces stockouts, and ensures continuous readiness.
Moreover, AI-driven platforms are increasingly acting as intermediaries between robotic clients and pharmaceutical vendors. These platforms use predictive analytics to forecast drug usage, monitor expiry dates, and automate prescription refills. The result is a healthcare procurement ecosystem where machines not only serve but also sustain themselves through intelligent resource acquisition.
Agriculture: Autonomous Machines Managing Inputs
The agricultural sector is undergoing rapid transformation through the deployment of autonomous farming equipment capable of seeding, harvesting, fertilizing, and soil monitoring with minimal human intervention. These machines are now also participating in automated input procurement, acquiring seeds, nutrients, fuel, and equipment parts based on algorithmic assessments of crop cycles, environmental data, and yield forecasts.
For instance, autonomous tractors integrated with satellite imagery and IoT-based soil sensors can evaluate the need for specific crop varieties or fertilizers, compare vendor offerings, and place orders through digital supply platforms. These transactions are executed with consideration of climate forecasts, regional price trends, and past performance data, enabling machines to act not only as laborers but also as strategic procurement agents.
This evolution supports the rise of precision agriculture, where every input is optimized to specific geospatial and temporal parameters. Vendors serving this sector are increasingly developing machine-compatible interfaces, subscription-based input delivery models, and usage-based billing systems to accommodate robotic buyers.
In this context, robotic clients are becoming not just consumers of agricultural inputs but also orchestrators of agroeconomic decisions, influencing demand signals, pricing models, and sustainability metrics across the agricultural supply chain.
Logistics and Transportation: Self-Servicing Delivery Networks
The logistics and transportation sector, traditionally reliant on manual coordination, is becoming increasingly autonomous through the adoption of self-navigating vehicles, drones, and robotic handling systems. These platforms are not only executing tasks but also initiating transactions necessary for their continued operation.
Delivery drones may purchase airspace licenses, maintenance services, or battery replacements based on usage metrics. Autonomous freight vehicles may initiate fueling or charging subscriptions, negotiate toll payments, and manage route optimization services using real-time traffic data. This creates a decentralized logistics network in which vehicles function as independent economic agents capable of self-servicing through transactional automation.
Companies like Starship Technologies and Nuro have begun to implement models where delivery robots automatically manage their own maintenance schedules and inventory replenishment for onboard tools or consumables. Fleet management software facilitates M2M procurement by analyzing operational metrics and triggering distributed purchases on behalf of individual robotic units.
As this trend matures, we may see the development of marketplaces specifically designed for autonomous vehicles, offering modular services such as navigation data, spare parts, performance analytics, and environmental compliance—each of which can be autonomously procured by robotic clients.
Legal, Ethical, and Governance Considerations
As robots expand their roles as economic actors across sectors, pressing legal and ethical questions arise. Who is liable if a robotic client makes an erroneous or harmful purchase? How are contractual obligations enforced when one party is non-human? These questions highlight the need for clear regulatory frameworks and governance structures that can accommodate non-traditional clients.
In many jurisdictions, legal personhood is a prerequisite for contract enforcement. While some corporations have advocated for limited economic agency status for robotic systems, there remains significant ambiguity. Without formal recognition, the enforceability of machine-executed agreements may be challenged, particularly in cross-border transactions or high-stakes contracts.
Ethically, the deployment of robotic clients also raises concerns about market fairness and systemic risk. Autonomous agents operating at scale could manipulate pricing dynamics, hoard critical resources, or execute high-frequency transactions that disadvantage smaller market participants. Regulators must consider these implications when designing policies that govern robotic participation in commercial ecosystems.
Additionally, the question of data governance becomes critical. Robotic clients require access to vast quantities of structured and unstructured data to make informed purchasing decisions. Vendors and platform providers must ensure that data privacy, usage rights, and security standards are upheld to prevent misuse or data exploitation.
Interoperability and the Emergence of Machine-Centric Standards
To facilitate cross-sector robotic commerce, industry-wide standards and interoperability frameworks are essential. Current procurement systems are often siloed, proprietary, and designed with human users in mind. As machines become the dominant participants in certain transactional workflows, there is a growing need for machine-first protocols.
Initiatives are underway to establish open-source standards for robotic procurement interfaces, digital identity verification, and machine-readable SLAs. These standards aim to ensure that autonomous clients can seamlessly operate across vendors, platforms, and regulatory environments without requiring custom integration or manual intervention.
Furthermore, interoperability enables the emergence of multi-sector robotic ecosystems, where a healthcare diagnostic bot can procure computing resources from a data center, which in turn sources energy from a robotic grid optimizer. This web of inter-machine commerce forms the foundation of a self-regulating machine economy, characterized by resilience, responsiveness, and scale.
In summary, the expansion of robots as clients into finance, healthcare, agriculture, and logistics illustrates the universality and adaptability of autonomous commerce. These machines are not confined by verticals—they operate wherever transactional logic, data, and machine agency converge. As organizations across sectors embrace this shift, they must rethink how services are packaged, how contracts are structured, and how clients are defined. The era of the robotic customer is not a retail anomaly—it is a systemic transformation, one that is poised to redefine commerce as we know it.
The Road Ahead: Opportunities and Challenges
As robots increasingly function as autonomous clients across retail and adjacent sectors, it is evident that their role is not a fleeting technological experiment but a structural evolution with enduring implications. The growing presence of robotic buyers introduces a fundamentally new customer class—intelligent, automated, and data-driven—that requires a radical rethinking of enterprise systems, commercial strategies, and regulatory paradigms. Looking ahead, both opportunities and challenges will shape the trajectory of this transformation, demanding a holistic response from businesses, technologists, and policymakers alike.
Opportunities: Unlocking a New Class of Economic Actors
The rise of robots as clients represents one of the most promising frontiers for enterprise value creation. These autonomous systems offer unparalleled efficiency, reliability, and scalability as customers. Because they operate based on logic rather than sentiment, robotic buyers make decisions faster, with greater precision, and with minimal variance. This allows businesses to forecast demand more accurately, optimize fulfillment, and reduce the costs associated with sales cycles and customer service.
Furthermore, robotic clients can operate continuously, enabling round-the-clock transactional ecosystems. Unlike human consumers who purchase within time-bound cycles, robots can initiate purchases at any hour, based on real-time inputs and operational triggers. This leads to a more fluid and continuous commercial rhythm, where traditional downtime is eliminated and revenue opportunities are expanded.
Another major opportunity lies in hyper-personalization and data feedback loops. Robotic clients generate rich datasets about their transactional behaviors, operational contexts, and supplier interactions. Vendors can analyze these datasets to fine-tune their offerings, streamline delivery schedules, and preemptively resolve integration issues. This feedback-driven model enables highly customized products and services designed specifically for robotic consumption, from modular APIs to machine-readable documentation and predictive maintenance protocols.
In addition, the emergence of machine-to-machine (M2M) commerce has the potential to give rise to entirely new business models. For instance, autonomous manufacturing equipment could subscribe to consumables as-a-service; robotic agricultural platforms might pay-per-use for sensor data or weather models; and delivery drones could dynamically purchase access to prioritized air corridors. These scenarios illustrate a future where robots are not just clients but autonomous nodes in decentralized economic networks, participating in transactions with other machines based on shared logic and programmable incentives.
Infrastructure and Ecosystem Expansion
To capitalize on these opportunities, companies must invest in robust digital infrastructure capable of supporting robotic clients at scale. This includes not only high-availability APIs and low-latency transaction platforms, but also autonomous monitoring tools, digital identity systems, and adaptive pricing engines. Just as the e-commerce boom of the early 2000s required a complete overhaul of retail logistics and web architecture, the robot client era will demand a new generation of machine-centric commerce infrastructure.
Enterprises should also consider the strategic value of robotic client partnerships, wherein vendors work directly with manufacturers of robotic platforms to pre-integrate offerings or provide embedded purchasing pathways. These partnerships can generate long-term revenue streams by locking in robotic clients over multiple procurement cycles through technical compatibility and performance reliability.
Challenges: Governance, Accountability, and Ethical Complexity
Despite the promising outlook, the robot-as-client paradigm introduces a series of complex challenges that must be addressed for sustainable adoption. Among the most pressing is the issue of accountability. When a robot makes a purchase or engages in a contractual agreement, determining liability in the event of a dispute or malfunction becomes a legal and ethical gray area. If a robotic system overorders, selects a defective supplier, or violates terms due to a software bug, who is responsible—the manufacturer, the software developer, the system integrator, or the enterprise that deployed it?
This question becomes more acute in high-stakes industries such as healthcare, defense, or energy, where erroneous purchases or faulty service contracts could lead to significant harm. Current legal frameworks do not adequately define the status of robotic clients as contracting parties, nor do they clarify the rights and responsibilities of the human entities behind them. Without clear regulations, the risk of litigation and reputational damage may deter organizations from fully embracing autonomous purchasing systems.
Closely related to accountability is the issue of trust and verification. Vendors interacting with robotic clients must be able to verify the identity, credentials, and authority of these systems to engage in transactions. This will require the development of digital identity protocols for machines, complete with cryptographic verification, access control, and audit logging. Emerging technologies such as decentralized identity (DID) frameworks and blockchain-based smart contracts may offer potential solutions, but widespread implementation remains in early stages.
Cybersecurity and Resilience Risks
The increased autonomy and integration of robotic clients also introduce heightened cybersecurity risks. Because these systems often operate autonomously and at high frequency, they present attractive targets for exploitation. A compromised robotic client could be manipulated to make unauthorized purchases, disrupt supply chains, or expose sensitive transactional data. Moreover, because robotic clients are often interconnected through networks of vendors, APIs, and fulfillment platforms, a single breach could propagate rapidly through the ecosystem.
Businesses must therefore treat robotic clients as critical infrastructure within their digital supply chains. End-to-end encryption, behavioral anomaly detection, real-time monitoring, and robust fail-safe protocols must be implemented to protect against manipulation and ensure system resilience. Cybersecurity strategies should also account for adversarial AI techniques, which could be used to confuse or mislead robotic decision-making algorithms.
Additionally, resilience planning must include fallback mechanisms to ensure continuity in the event of robotic system failure. For example, human intervention triggers, backup procurement routes, and manual override capabilities should be in place to prevent operational disruptions when robotic clients are disabled or impaired.
Economic Displacement and Human Workforce Implications
While the rise of robotic clients creates new efficiencies, it also introduces questions around economic displacement and workforce transformation. Roles traditionally associated with purchasing, vendor management, and supply chain coordination may be partially or fully automated. As these functions become algorithmically driven, the human labor force must adapt by acquiring new skills in robotic systems integration, data analytics, and machine interface design.
Organizations must proactively address this shift through reskilling programs and cross-functional training, ensuring that displaced roles are not simply eliminated but redefined. There will be growing demand for professionals who can manage hybrid client ecosystems composed of both human and robotic actors, interpret machine-generated data, and maintain digital commerce infrastructure.
Moreover, the displacement effect may not be limited to internal roles. Third-party service providers—such as call centers, procurement consultants, and account managers—may also face obsolescence unless they reposition their services to offer higher-value, automation-enhancing solutions.
Long-Term Societal and Regulatory Considerations
In the long term, the proliferation of robotic clients may necessitate a societal redefinition of commerce, autonomy, and agency. As machines become participants in economic systems, issues of taxation, antitrust, and consumer rights may be re-evaluated. Should robotic clients be taxed as consumers? Can they form collectives or associations? Are there ethical limits to the autonomy they should possess?
Regulators must begin to craft machine commerce policy frameworks that anticipate future developments while ensuring equitable market participation and societal benefit. Such frameworks should be designed collaboratively with industry stakeholders, technologists, ethicists, and policymakers to strike a balance between innovation and public interest.
In summary, the road ahead for robots as clients is filled with promise and complexity. The opportunities for efficiency, growth, and innovation are immense, but they are counterbalanced by challenges that span law, ethics, cybersecurity, and workforce dynamics. To realize the full potential of this transformation, stakeholders must engage with foresight, adaptability, and a commitment to building resilient, inclusive, and ethically grounded systems. The robotic client is not just a tool—it is a new actor in the global economy, and its influence will only continue to expand.
Conclusion
The ascent of robots as top clients in retail and beyond represents a paradigm shift in how businesses conceive of, engage with, and serve their customers. No longer limited to performing isolated tasks or supporting human workflows, robotic systems are emerging as autonomous participants in economic ecosystems, capable of initiating transactions, managing procurement cycles, and optimizing operational expenditures without direct human input.
Throughout this exploration, we have observed how robotic clients are reshaping multiple sectors—from the precise inventory management of retail and the intelligent capital allocation in finance, to the self-replenishing logistics operations and predictive procurement behaviors in agriculture and healthcare. Their rise introduces a new standard of performance, predictability, and programmability in commerce—one that challenges legacy systems and compels organizations to adapt swiftly.
For businesses, the implications are profound. Serving robotic clients demands a reconfiguration of supply chains, digital infrastructure, service-level agreements, and customer engagement models. Vendors must shift from persuasion-based sales tactics to performance-based integrations, offering machine-readable interfaces, high-reliability APIs, and scalable automation strategies. At the same time, the emergence of M2M commerce and decentralized machine economies opens up transformative opportunities for innovation and growth.
Yet, these opportunities come with challenges that must not be underestimated. Issues surrounding liability, cybersecurity, workforce displacement, and regulatory ambiguity require urgent attention. As robotic clients continue to proliferate, cross-disciplinary collaboration between technologists, policymakers, business leaders, and ethicists will be essential to ensure that this evolution is both sustainable and equitable.
In conclusion, the rise of robots as clients is not a fringe development—it is a structural transition toward a machine-integrated economy. Organizations that recognize and respond to this shift today will gain a competitive advantage in a future where commerce is increasingly driven not by human hands or voices, but by autonomous logic, data, and digital intent. The future of the customer is not only human—it is robotic, and it has already arrived.
References
- OpenAI. "Robots as Economic Agents: Multi-Agent Simulations and Market Behaviors."
https://openai.com/research/multi-agent-emergent-behaviors - Amazon Web Services. "Build M2M Commerce Systems with Serverless APIs."
https://aws.amazon.com/blogs/apn/building-machine-to-machine-commerce-with-serverless - JD.com. "Fully Automated Warehouses and the Future of Smart Retail."
https://corporate.jd.com/newsDetail - McKinsey & Company. "The Autonomous Supply Chain: Shifting from Reactive to Predictive."
https://www.mckinsey.com/business-functions/operations/our-insights/autonomous-supply-chain - NVIDIA. "AI-Powered Robots: Redefining Retail and Warehousing Operations."
https://blogs.nvidia.com/blog/robotics-retail-ai - IBM. "The Role of AI in Procurement: Automating Supply Chains with Cognitive Agents."
https://www.ibm.com/blogs/insights-on-business/ai-procurement-automation - World Economic Forum. "Machines as Consumers: Preparing for the Age of the Robotic Buyer."
https://www.weforum.org/agenda/robot-buyers-future-commerce - Microsoft Azure. "Empowering Autonomous Systems with Cloud-Driven AI."
https://azure.microsoft.com/en-us/blog/autonomous-systems-cloud-ai - BCG. "The Next Digital Frontier: Serving Non-Human Clients."
https://www.bcg.com/publications/digital-transformation-machine-clients - Accenture. "The Machine Economy: How Companies Can Adapt to Intelligent Digital Buyers."
https://www.accenture.com/us-en/insights/technology/machine-economy