Driving the Future: Autonomous Vehicles, AI Technology, and NVIDIA’s Pivotal Role
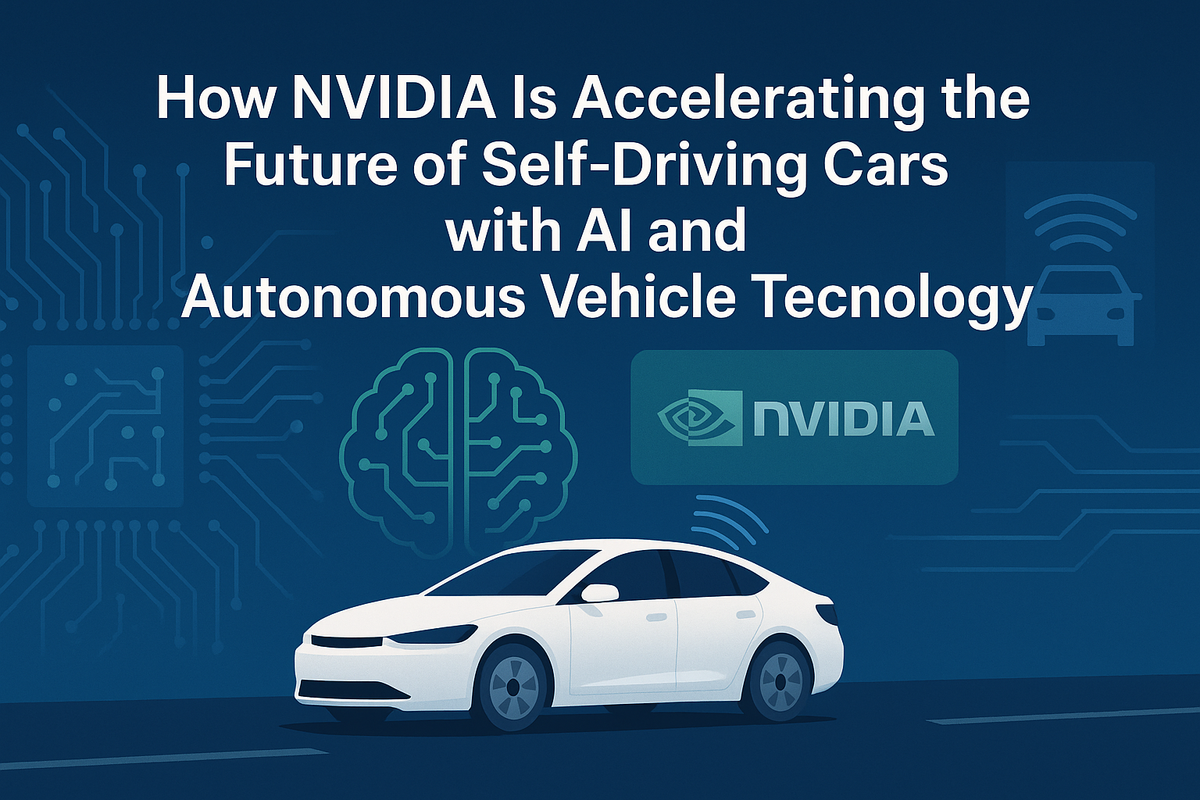
The global transportation sector is undergoing a seismic shift, driven by advancements in artificial intelligence (AI) and autonomous vehicle (AV) technologies. From early research prototypes to today’s semi-autonomous systems navigating urban streets, the evolution of driverless cars has moved from the realm of science fiction into tangible reality. At the heart of this transformation is a sophisticated interplay of sensors, algorithms, and machine learning models—all functioning together to enable vehicles to perceive, interpret, and interact with their environments in real time.
The promise of autonomous vehicles extends far beyond technological novelty. These systems have the potential to drastically reduce traffic accidents caused by human error, improve traffic efficiency, reduce carbon emissions through optimized driving, and expand mobility access for populations previously underserved by traditional transportation. As urban populations grow and mobility demands intensify, the appeal of safe, efficient, and AI-driven transportation solutions has captured the attention of governments, automakers, and technology companies alike.
Among the leaders at the forefront of this revolution is NVIDIA, a company historically known for its graphics processing units (GPUs) and now widely recognized as a pivotal player in the development of intelligent vehicle systems. NVIDIA's contributions to AV development span across hardware acceleration, software frameworks, and virtual testing environments—each a critical piece of the autonomous vehicle puzzle.
This blog post explores the layered world of autonomous vehicles and AI, delving into the core technologies that make autonomy possible, the trajectory of AV development over the past two decades, and the transformative role NVIDIA continues to play in shaping the future of mobility. Through detailed analysis, real-world case studies, and data-driven insights, we aim to illuminate how AI and advanced computing are redefining the automotive landscape—and what this means for consumers, industries, and global infrastructure in the years ahead.
The Evolution of Autonomous Vehicles
The development of autonomous vehicles (AVs) represents one of the most significant technological undertakings in modern history. From early conceptualizations to today’s sophisticated systems capable of navigating complex environments, the journey of AV technology reflects decades of interdisciplinary innovation. Understanding this evolution requires examining the progression of autonomy levels, pivotal milestones in research and development, and the leading players who have contributed to the advancement of this dynamic field.
Defining Autonomy: SAE Levels 0 to 5
The Society of Automotive Engineers (SAE) has standardized autonomy into six distinct levels, ranging from Level 0 (no automation) to Level 5 (full automation). Each level demarcates the increasing capabilities of vehicles to perform driving tasks with minimal to no human intervention.
- Level 0: No automation. The human driver performs all driving tasks.
- Level 1: Driver assistance. Features such as adaptive cruise control or lane-keeping assist are available, but the driver must remain engaged.
- Level 2: Partial automation. The vehicle can control steering and acceleration/deceleration simultaneously, yet the driver must monitor the environment.
- Level 3: Conditional automation. The vehicle can handle driving in specific conditions, and the driver can disengage but must be ready to intervene if needed.
- Level 4: High automation. The vehicle can perform all driving tasks within defined geofenced areas without human oversight.
- Level 5: Full automation. The vehicle is capable of autonomous operation under all conditions without any human input.
This framework has enabled clearer regulatory guidance and market communication, fostering alignment among automakers, regulators, and consumers.
Pioneering Efforts and Technological Milestones
The concept of autonomous driving dates back to the 1920s, when early demonstrations of "driverless" cars used radio signals to control basic functions. However, substantive progress did not emerge until the 1980s, when academic institutions and government agencies began investing in computer vision and robotics research. Carnegie Mellon University’s Navlab and Mercedes-Benz’s VaMoRs project were early attempts to build self-driving prototypes capable of limited autonomous navigation.
The real inflection point came in the early 2000s with the introduction of the DARPA Grand Challenge, organized by the U.S. Department of Defense. The inaugural 2004 competition saw no team complete the course, underscoring the immaturity of the technology. Yet, by 2005, five vehicles completed a 132-mile desert course autonomously, with Stanford University’s vehicle taking first place. These competitions catalyzed a generation of AV startups and academic research groups, many of which evolved into today’s leading AV firms.
Subsequent advances in AI, particularly the advent of deep learning, revolutionized perception and decision-making capabilities. The 2010s witnessed exponential growth in the field, with companies like Google launching their self-driving car project (now Waymo), Tesla introducing Autopilot for consumer vehicles, and Uber and Lyft exploring autonomous ride-hailing services. Today, AV pilot programs operate in multiple cities worldwide, supported by robust AI infrastructures and vast datasets collected from millions of miles of real-world and simulated driving.
Commercialization and Industry Leaders
The race toward fully autonomous vehicles has become a multi-billion-dollar endeavor involving a diverse ecosystem of players. These include traditional automakers, technology giants, and highly specialized startups.
- Waymo (Alphabet): A pioneer in AV technology, Waymo has logged millions of miles on public roads and offers fully autonomous ride-hailing services in Phoenix, Arizona.
- Tesla: Leveraging its consumer vehicle base, Tesla has deployed a vision-based Full Self-Driving (FSD) system in beta, aiming to achieve autonomy without LiDAR.
- Cruise (GM): Operating robotaxis in San Francisco, Cruise is backed by General Motors and Honda, focusing on dense urban mobility solutions.
- Mobileye (Intel): Originally focused on advanced driver-assistance systems (ADAS), Mobileye has expanded into full AV development with a scalable AV platform.
- Baidu Apollo: In China, Baidu’s Apollo project has developed autonomous taxis and buses, becoming a key player in Asia’s AV ecosystem.
- Aurora Innovation, Zoox, Pony.ai, and Nuro: These startups represent a new wave of AV ventures focused on logistics, urban ride-hailing, and last-mile delivery.
This competitive landscape has accelerated innovation, resulting in faster iteration cycles, expanded deployment scenarios, and greater consumer exposure to AV technologies.
Technological Dependencies and Interdisciplinary Fusion
Autonomous vehicles rely on an intricate combination of technologies. Unlike traditional automotive systems, AVs integrate disciplines such as robotics, machine learning, high-definition mapping, sensor fusion, and systems engineering. Each core function—perception, localization, planning, and control—depends on finely tuned AI models trained on petabytes of data.
Perception systems use a mix of LiDAR, radar, and cameras to detect and classify objects. Localization modules determine the vehicle's exact position, often with centimeter-level precision, using GPS data and real-time environmental mapping. Path planning algorithms chart safe, efficient routes through dynamic environments, while control systems execute the driving commands with millisecond-level feedback loops.
Crucially, these modules must operate in real time under stringent safety constraints. Ensuring reliability across diverse weather conditions, traffic patterns, and geographic settings remains a core challenge in scaling AV deployment. As a result, massive investments in simulation platforms and synthetic data generation have emerged as necessary complements to on-road testing.
This timeline visualizes the key breakthroughs from early research through to modern deployments:
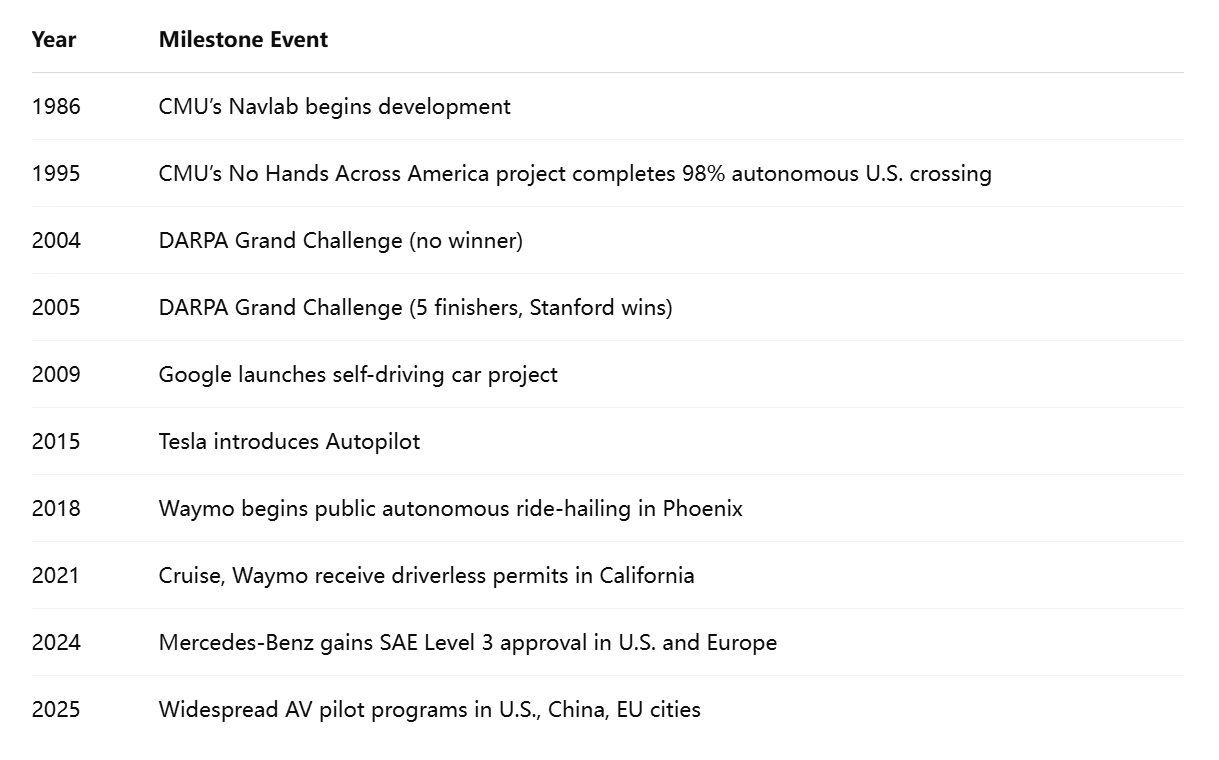
This chronology underscores the accelerated progress of AV technology in the past two decades, driven by both public research and private investment.
Conclusion
The evolution of autonomous vehicles is the result of decades of technological convergence. From DARPA challenges to real-world deployments, the development of AVs has matured into a sophisticated, interdisciplinary endeavor. As we progress toward higher levels of autonomy, the role of AI continues to grow, necessitating advancements not only in algorithms but also in computing hardware, sensor technologies, and regulatory frameworks. With multiple global stakeholders now involved, the journey toward safe, scalable, and economically viable autonomy is well underway.
AI Technologies Powering Autonomous Vehicles
The cornerstone of modern autonomous vehicles (AVs) is artificial intelligence (AI). While the mechanical engineering and sensor components of self-driving cars are critical, it is AI that empowers vehicles to interpret the world, make decisions, and navigate complex and dynamic environments. The convergence of machine learning, neural networks, computer vision, sensor fusion, and simulation technologies has revolutionized the capabilities of AV systems. In this section, we examine the core AI technologies that power autonomous vehicles and explore how these systems interact to produce safe, efficient, and intelligent driving behaviors.
Machine Learning and Deep Learning Foundations
At the heart of autonomous driving lies machine learning, a subset of AI that enables systems to improve their performance through experience. More specifically, deep learning—a type of machine learning based on artificial neural networks—has become the dominant paradigm in AV development due to its unparalleled ability to process large volumes of data and identify complex patterns.
Deep learning models are used in a range of AV functions, from detecting traffic signs to predicting pedestrian movements. These models are trained on vast datasets that include camera images, LiDAR point clouds, radar signals, and vehicle telemetry. Convolutional Neural Networks (CNNs), for example, are highly effective in processing visual data from cameras, enabling the AV to recognize traffic lights, road signs, lane markings, and other vehicles.
Recurrent Neural Networks (RNNs) and their more sophisticated variant, Long Short-Term Memory (LSTM) networks, are often employed in motion prediction and behavioral modeling. These models consider temporal data and allow the AV to anticipate the future positions of dynamic agents such as cyclists and pedestrians based on their movement history.
Computer Vision and Perception
Computer vision is the AI subfield that enables machines to interpret and understand visual information. In AVs, computer vision is crucial for object detection, semantic segmentation, depth estimation, and scene understanding. Cameras mounted around the vehicle continuously capture high-resolution images, which are then processed to identify objects and determine their spatial relationships.
Object detection models such as YOLO (You Only Look Once) and SSD (Single Shot Detector) are commonly used to identify and localize objects like vehicles, traffic signals, and pedestrians. Semantic segmentation models go a step further by labeling each pixel in an image, enabling the system to distinguish between the road, sidewalks, buildings, and other elements.
Depth estimation, either through stereo vision or monocular cues augmented by neural networks, helps the AV understand the three-dimensional structure of its environment. Combined, these visual processing capabilities allow the vehicle to form a detailed and dynamic model of the world around it—essential for safe navigation.
Sensor Fusion: Integrating Multiple Data Streams
While cameras are critical for perception, they are not sufficient on their own. AVs use a multi-modal sensor suite that typically includes LiDAR, radar, ultrasonic sensors, and GPS. Sensor fusion is the process of integrating data from these disparate sources to create a unified and accurate model of the surrounding environment.
LiDAR (Light Detection and Ranging) provides high-resolution 3D maps by measuring the time it takes for laser pulses to reflect off objects. This technology is particularly useful for detecting the precise shape and location of nearby objects and is resilient in various lighting conditions.
Radar, which uses radio waves, is effective for detecting objects at long ranges and in poor visibility conditions such as fog, rain, or snow. It complements LiDAR by offering reliable velocity and distance measurements.
Ultrasonic sensors assist with near-field object detection, particularly useful for low-speed maneuvers like parking. GPS and Inertial Measurement Units (IMUs) provide geospatial awareness, enabling localization with respect to high-definition (HD) maps.
AI-driven sensor fusion algorithms combine these data streams in real time, improving robustness and redundancy. For instance, if a camera’s view is obstructed by glare, the AV can still rely on LiDAR and radar to maintain situational awareness. This redundancy is vital for safety and reliability, especially in unstructured or unpredictable environments.
Localization and Mapping
Accurate localization is essential for autonomous vehicles to understand their position within the broader environment. This is typically achieved through simultaneous localization and mapping (SLAM) techniques, which allow the vehicle to build and update maps while keeping track of its own location.
AVs often use high-definition maps that include detailed information about road geometry, traffic signals, stop signs, and other static elements. Localization systems compare real-time sensor data against these maps to estimate the vehicle’s exact position—often within a few centimeters of accuracy.
AI enhances localization through techniques such as particle filters and Kalman filters, which probabilistically estimate the vehicle’s position by accounting for uncertainty in sensor measurements. More advanced approaches, such as deep learning-based visual odometry, allow for accurate localization without relying heavily on pre-mapped environments.
Path Planning and Decision-Making
Once an AV perceives its surroundings and localizes itself on the map, it must determine the safest and most efficient path to its destination. This involves two primary AI functions: path planning and decision-making.
Path planning includes both global planning (finding the optimal route from origin to destination) and local planning (navigating around obstacles, obeying traffic laws, and responding to dynamic changes). Algorithms such as A*, RRT* (Rapidly-exploring Random Tree Star), and Dijkstra’s algorithm are widely used for global route planning.
For real-time decision-making, AVs rely on a combination of rule-based systems and learning-based models. Reinforcement learning, a branch of machine learning, is particularly valuable in this context. In reinforcement learning, an agent learns optimal driving behaviors through trial and error within simulated environments. This approach enables AVs to develop complex policies for merging lanes, yielding to pedestrians, and handling edge cases that rule-based systems may not adequately address.
Behavior prediction is another vital aspect. AI models forecast the actions of surrounding agents—such as whether a pedestrian is likely to cross the road or whether another vehicle will change lanes. Accurate predictions are crucial for safe interaction in shared spaces.
Simulation and Synthetic Data
Given the vast number of scenarios AVs must handle, physical testing alone is insufficient. Simulation plays a vital role in training and validating AI systems. Modern AV development environments rely on advanced simulation platforms that can recreate urban traffic, weather conditions, and road infrastructure with high fidelity.
Companies use platforms such as NVIDIA DRIVE Sim, Carla, and LGSVL to conduct thousands of virtual driving hours in conditions that would be impractical or unsafe to test on public roads. These simulators generate synthetic data used to train deep learning models, including rare and dangerous edge cases.
Synthetic data also helps overcome the limitations of real-world data collection. For example, training an AV to respond to a deer crossing the road at night in foggy conditions is a challenge due to the rarity of such events. Simulation allows developers to create and annotate these scenarios, ensuring the AI is prepared for real-world unpredictability.
AI Safety, Explainability, and Regulatory Compliance
As AVs gain more autonomy, ensuring the safety and transparency of AI systems becomes increasingly critical. AI safety focuses on building systems that are robust to errors, adversarial inputs, and hardware failures. Techniques such as redundancy, fail-safe mechanisms, and continuous monitoring are essential components of safety-critical AV systems.
Explainable AI (XAI) aims to provide transparency into how AI models make decisions. This is especially important in scenarios where an AV is involved in a collision or unexpected behavior. Regulatory bodies may require documentation or justifications for the system's actions, necessitating interpretable models or visualizations that can reconstruct decision pathways.
Additionally, autonomous driving systems must comply with local traffic laws, data privacy regulations, and cybersecurity standards. As regulatory frameworks evolve, AV companies are incorporating governance and compliance modules directly into their AI stacks.
Conclusion
The deployment of autonomous vehicles is fundamentally an AI-driven endeavor. From perception and localization to planning and control, AI technologies orchestrate a vehicle's understanding of and interaction with its environment. These systems are made possible through innovations in deep learning, computer vision, sensor fusion, and simulation. As AVs move closer to mainstream adoption, the continued advancement of these technologies—and their integration into scalable, safe, and transparent platforms—will determine the pace and scope of transformation across the transportation industry.
NVIDIA’s End-to-End Autonomous Vehicles Technology Stack
NVIDIA has evolved from a leader in high-performance graphics to a pivotal force in the development of autonomous vehicle (AV) technologies. Through a strategic combination of purpose-built hardware, comprehensive software platforms, and robust simulation environments, NVIDIA offers one of the most integrated and scalable AV ecosystems in the industry. This end-to-end stack not only supports real-time perception, planning, and control but also addresses the broader challenges of data management, training, and simulation. In this section, we examine NVIDIA’s full-stack AV architecture and its critical role in enabling safe and intelligent autonomous driving.
The Hardware Foundation: From GPUs to System-on-Chips
NVIDIA’s success in autonomous driving is rooted in its ability to deliver high-performance computing power in compact and energy-efficient formats. The company’s hardware offerings range from general-purpose graphics processing units (GPUs) to automotive-grade system-on-chips (SoCs) specifically designed for the complex workloads of autonomous driving.
NVIDIA DRIVE AGX
NVIDIA DRIVE AGX is the foundational hardware platform for autonomous vehicles. It combines NVIDIA’s Xavier and Orin SoCs to provide the computing capacity necessary for processing sensor data, running deep learning models, and executing control algorithms—all in real time. DRIVE AGX supports SAE Levels 2 through 5 of vehicle autonomy and is built to withstand the rigorous safety and reliability requirements of the automotive industry.
- Xavier: Capable of delivering 30 TOPS (trillions of operations per second), Xavier was the first SoC to be designed specifically for autonomous driving, integrating a GPU, CPU, and deep learning accelerator.
- Orin: With performance up to 254 TOPS, Orin supports higher levels of autonomy and offers 7x the performance of Xavier. It supports multiple concurrent deep neural networks and real-time data fusion from up to 12 cameras, 9 radars, and 12 ultrasonic sensors.
NVIDIA DRIVE Thor
Launched as the next-generation automotive-grade SoC, DRIVE Thor is designed to unify autonomous driving and infotainment systems into a single architecture. Offering up to 2000 TOPS of processing power, Thor is intended to replace multiple electronic control units (ECUs), simplifying system integration and reducing cost and complexity. Its inclusion of a Transformer Engine makes it particularly well-suited for running generative AI models and next-generation large-scale networks.
Software Infrastructure: DRIVE OS, DRIVE AV, and DRIVE IX
NVIDIA complements its hardware platforms with a robust suite of software that handles perception, mapping, planning, and user experience.
DRIVE OS
DRIVE OS is the foundational operating system for NVIDIA’s automotive platforms. Built on a real-time Linux kernel, DRIVE OS provides low-level support for scheduling, memory management, and sensor data processing. It includes safety libraries compliant with ISO 26262 and supports the use of CUDA, TensorRT, and other NVIDIA acceleration tools. DRIVE OS acts as the bridge between hardware and higher-level AV functions, ensuring optimal resource utilization and deterministic behavior.
DRIVE AV
NVIDIA DRIVE AV is a modular software stack for autonomous driving. It includes components for sensor fusion, localization, perception, prediction, planning, and control. Each module is designed to be interoperable, allowing automakers to integrate NVIDIA’s solutions with their proprietary systems or third-party tools. Key features include:
- Perception: AI-powered object detection, lane segmentation, and drivable space recognition using fused data from cameras, LiDAR, and radar.
- Localization: High-precision localization through HD map matching and visual odometry.
- Prediction and Planning: Real-time behavioral prediction of surrounding agents and trajectory generation using cost-based optimization.
DRIVE AV is highly scalable and supports both supervised and unsupervised learning models, enabling continuous learning and improvement through fleet-wide data aggregation.
DRIVE IX
DRIVE IX (Intelligent Experience) focuses on the in-cabin experience, using AI to enhance interaction between the vehicle and its occupants. It supports driver monitoring, voice recognition, facial identification, and gesture control. The system is designed to improve both safety and personalization, adapting the vehicle environment based on driver behavior and preferences.
NVIDIA Omniverse and DRIVE Sim: The Virtual Testing Environment
Real-world testing of autonomous vehicles is limited by logistical, environmental, and safety constraints. To address these limitations, NVIDIA has invested heavily in simulation technologies—most notably NVIDIA Omniverse and DRIVE Sim.
NVIDIA Omniverse
Omniverse is a collaborative platform that allows developers to build physically accurate, photo-realistic virtual environments. It uses Universal Scene Description (USD) as a foundation and supports real-time synchronization across applications. For AV development, Omniverse enables the creation of digital twins of urban environments, which are critical for scenario planning, infrastructure modeling, and safety validation.
NVIDIA DRIVE Sim
DRIVE Sim is NVIDIA’s dedicated simulator for autonomous vehicle testing. Built on Omniverse, it supports high-fidelity simulation of sensors, environments, and traffic agents. Key capabilities include:
- Synthetic Data Generation: Generation of annotated datasets for training deep learning models in rare or hazardous scenarios.
- Scenario Testing: Replay of real-world events for diagnosis and validation.
- Hardware-in-the-Loop (HIL) Testing: Real-time interaction between simulation and physical hardware systems, allowing for comprehensive end-to-end validation.
DRIVE Sim supports closed-loop testing, enabling the continuous retraining and refinement of AI models using feedback from simulated experiences.
Collaborations and Industry Adoption
NVIDIA’s technology stack is not only comprehensive but also widely adopted across the automotive industry. The company has forged partnerships with leading OEMs, Tier 1 suppliers, and mobility startups to deliver production-ready AV solutions.
Mercedes-Benz
In collaboration with NVIDIA, Mercedes-Benz announced that all future vehicles will be built on the DRIVE AGX Orin platform. The integration supports Level 3 driving capabilities and advanced user interfaces. The partnership includes continuous over-the-air updates and cloud-to-car synchronization through NVIDIA’s AI infrastructure.
Volvo
Volvo has adopted NVIDIA’s technology to power its next-generation ADAS and autonomous systems, particularly in its Polestar and XC90 models. The partnership emphasizes safety, aligning with Volvo’s longstanding commitment to reducing traffic fatalities.
Hyundai and Genesis
Hyundai Motor Group has also integrated NVIDIA DRIVE platforms into its high-end models, aiming to bring AI-powered capabilities across infotainment, connectivity, and automated driving.
Startups and Robotaxi Platforms
NVIDIA’s stack is also used by mobility pioneers such as Zoox, Pony.ai, and TuSimple. These companies leverage DRIVE AGX and DRIVE Sim for development, simulation, and deployment of their respective ride-hailing and logistics solutions.
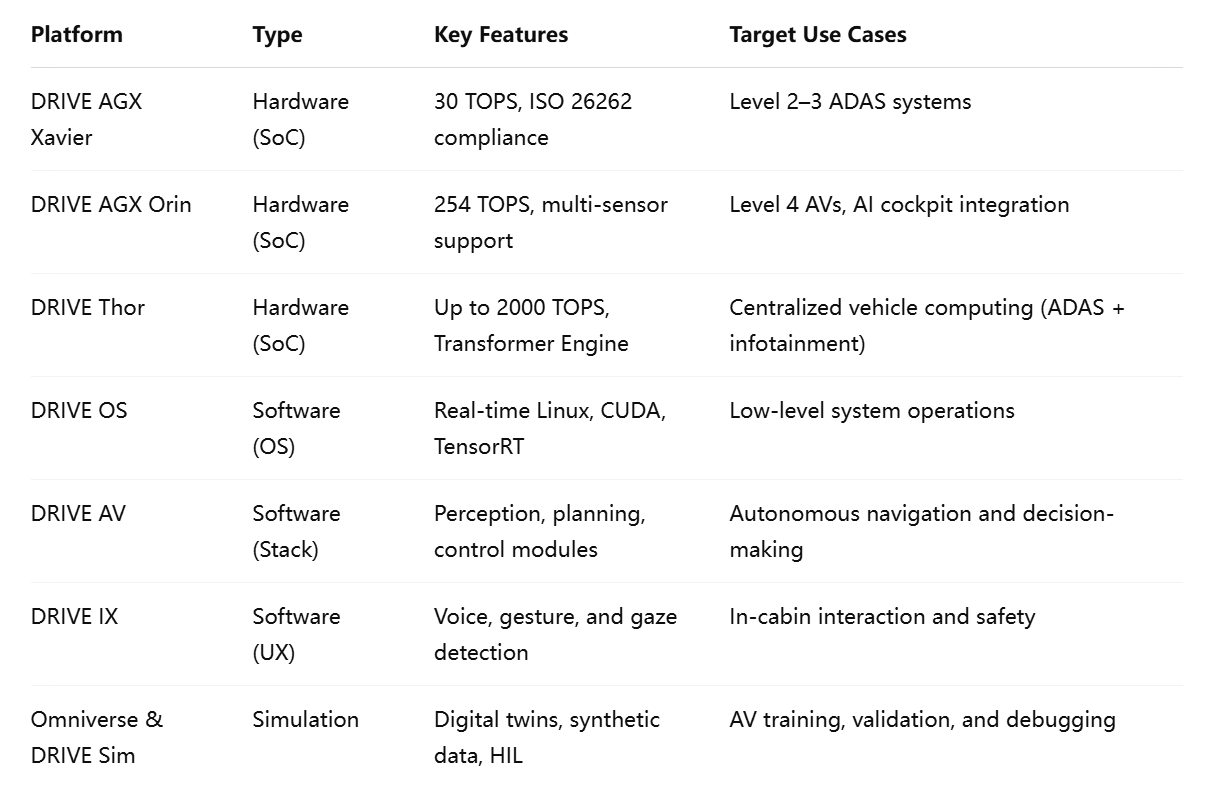
Conclusion
NVIDIA’s end-to-end AV stack exemplifies a vertically integrated approach to autonomous driving. By unifying powerful hardware, modular software, and advanced simulation environments, NVIDIA enables a wide array of partners to accelerate the development and deployment of autonomous systems. Its scalable architecture is designed not only to meet the computational demands of real-time AI processing but also to accommodate future innovations in generative AI and multi-agent simulation. As the AV industry matures, NVIDIA’s technology stack is positioned to serve as a central pillar in the ongoing evolution of intelligent mobility.
Real-World Applications and Industry Impact
The commercial deployment of autonomous vehicle (AV) technology has transitioned from experimental pilots to scalable applications in urban mobility, logistics, and smart infrastructure. With advancements in artificial intelligence (AI) and high-performance computing, AVs are now becoming operational in real-world scenarios—transforming business models, altering labor dynamics, and prompting new regulatory paradigms. At the heart of this transformation, NVIDIA’s hardware and software platforms play an instrumental role, serving as the digital engine behind many of today’s leading AV initiatives.
This section explores how NVIDIA-powered systems are being implemented across sectors and regions, highlights case studies of leading adopters, and presents an overview of the competitive landscape shaped by its technology.
The commercial deployment of autonomous vehicle (AV) technology has transitioned from experimental pilots to scalable applications in urban mobility, logistics, and smart infrastructure. With advancements in artificial intelligence (AI) and high-performance computing, AVs are now becoming operational in real-world scenarios—transforming business models, altering labor dynamics, and prompting new regulatory paradigms. At the heart of this transformation, NVIDIA’s hardware and software platforms play an instrumental role, serving as the digital engine behind many of today’s leading AV initiatives.
This section explores how NVIDIA-powered systems are being implemented across sectors and regions, highlights case studies of leading adopters, and presents an overview of the competitive landscape shaped by its technology.
Urban Mobility and Ride-Hailing
Autonomous ride-hailing is among the most prominent real-world applications of AV technology. Companies deploying robotaxi fleets are leveraging AI-powered systems for dynamic route planning, traffic interpretation, and real-time decision-making in densely populated environments. NVIDIA’s DRIVE AGX platform and DRIVE AV software have emerged as central components in several of these deployments.
Waymo and Cruise
Although Waymo primarily uses proprietary technology, NVIDIA’s computing infrastructure is utilized in its AI model training and simulation environments. Cruise, on the other hand, has adopted components of NVIDIA’s DRIVE platform to support its goal of launching fully driverless services in urban centers like San Francisco. By integrating NVIDIA’s high-performance AI accelerators and simulation capabilities, Cruise is able to iterate and validate its software stack more rapidly.
Pony.ai and Zoox
Pony.ai, operating in both the U.S. and China, uses NVIDIA’s DRIVE platform for perception and sensor fusion tasks. It runs its neural networks on NVIDIA GPUs both onboard and in the cloud, allowing real-time updates and over-the-air improvements. Similarly, Zoox, a subsidiary of Amazon, has incorporated NVIDIA’s simulation technologies to validate its bidirectional vehicle, designed for urban mobility with no steering wheel or driver's seat.
These robotaxi platforms demonstrate how NVIDIA’s scalable architecture supports not only real-time inferencing at the edge but also continuous AI training in the cloud, bridging the gap between development and deployment.
Logistics, Delivery, and Freight
The logistics industry has emerged as a high-impact sector for AV technology. From last-mile delivery robots to long-haul autonomous trucks, the use of AI to optimize goods movement is reshaping the global supply chain. NVIDIA has positioned itself as a key enabler in this domain through partnerships with companies that are redefining freight transport and automated delivery services.
TuSimple
TuSimple, a leader in autonomous freight transport, relies heavily on NVIDIA DRIVE for real-time perception and planning. Operating on long stretches of highway, TuSimple trucks use sensor fusion and AI decision-making to navigate complex scenarios such as lane changes, merging traffic, and construction zones. The company's adoption of DRIVE Orin ensures sufficient computational headroom for edge AI inference and future software upgrades.
Nuro
Nuro, which specializes in last-mile delivery using compact autonomous vehicles, uses NVIDIA’s GPUs for both onboard inference and offboard simulation. With commercial partnerships with companies like Domino’s and Kroger, Nuro’s fleet demonstrates how AI can support low-speed, high-frequency delivery in suburban environments. The company’s focus on safety, reliability, and operational efficiency aligns closely with NVIDIA’s core technological competencies.
Integration with Smart Cities and Infrastructure
Beyond individual vehicles, NVIDIA’s technologies are being integrated into broader urban mobility and infrastructure projects. As cities evolve into smart ecosystems, real-time data from AVs contribute to adaptive traffic management, emergency response optimization, and sustainability initiatives.
Digital Twins and Intelligent Intersections
Using NVIDIA Omniverse, cities and automotive companies are now building digital twins of urban environments. These virtual replicas allow stakeholders to simulate the effects of AV deployment at scale, analyze traffic flow under different conditions, and test emergency scenarios. Digital twins also facilitate collaboration between urban planners, automakers, and AI developers.
Moreover, NVIDIA is collaborating with municipalities and partners to develop intelligent intersections—urban crossroads equipped with edge AI systems that monitor pedestrian movement, bicycle traffic, and vehicle patterns. These systems can dynamically adjust signal timing to improve safety and reduce congestion, informed by the same perception algorithms used in autonomous vehicles.
Safety and Regulatory Compliance
NVIDIA’s AV platforms are engineered to meet the rigorous demands of safety certification and regulatory compliance. ISO 26262 compliance, functional safety libraries, and deterministic real-time operating systems are standard features of the DRIVE OS ecosystem. These elements are critical for deployment in regulated environments, where explainability and traceability are prerequisites for approval.
In the United States, the National Highway Traffic Safety Administration (NHTSA) and local departments of transportation have increasingly scrutinized AV deployments. NVIDIA’s investment in safety validation—especially through closed-loop simulation with DRIVE Sim—helps automakers and startups meet regulatory requirements more efficiently. In Europe and Asia, similar certification pathways are being pursued in partnership with NVIDIA to align AV deployments with region-specific standards.
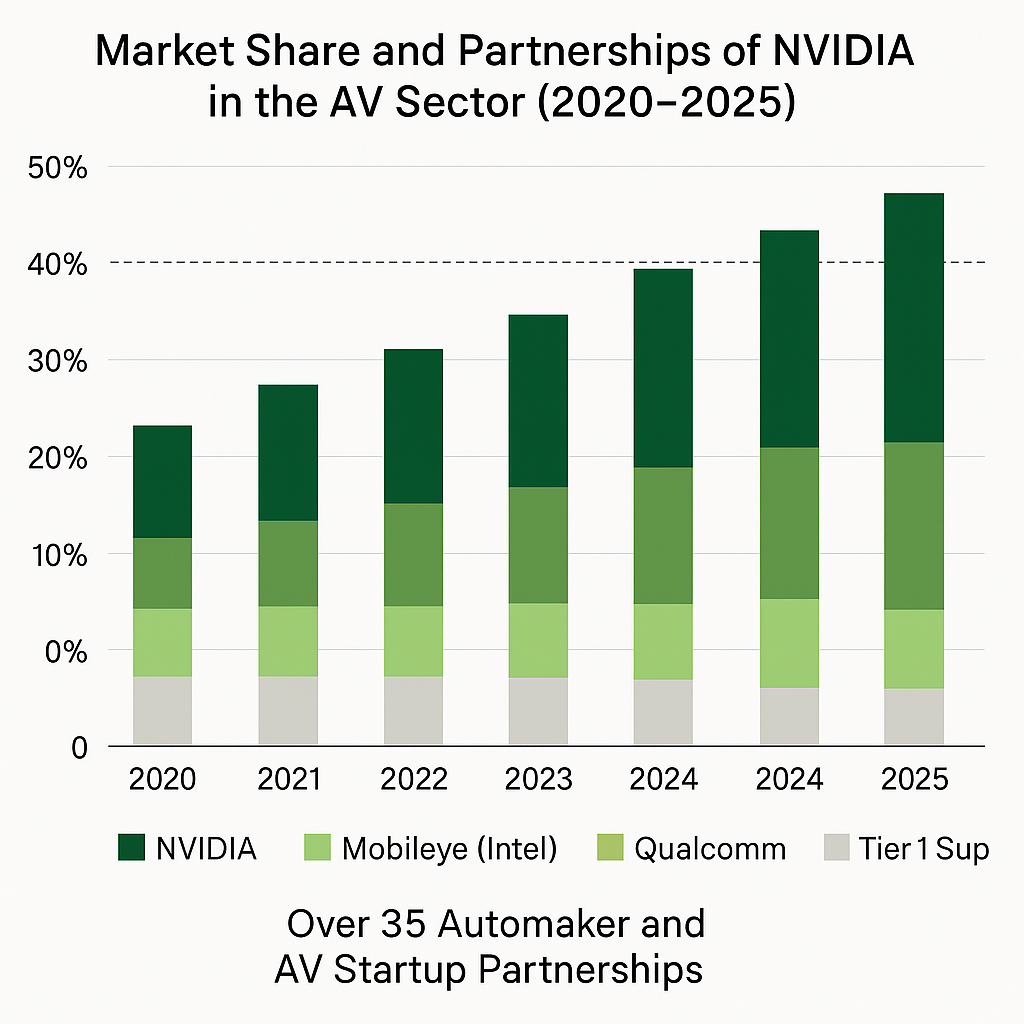
This chart underscores NVIDIA’s expanding footprint in the AV ecosystem, not only through product adoption but also via co-development and strategic alliances.
Conclusion
The real-world implementation of autonomous vehicles is no longer a theoretical endeavor. From dense urban centers to remote highway corridors, AV systems powered by AI—and enabled by NVIDIA’s full-stack solutions—are driving tangible progress across multiple industries. Through strategic collaborations, scalable hardware-software integration, and robust simulation capabilities, NVIDIA has emerged as a catalyst for the AV revolution. Its platforms empower a diverse array of stakeholders—from startups and logistics firms to smart city planners and traditional OEMs—to turn the vision of intelligent mobility into an operational reality.
Conclusion and Future Outlook
The convergence of artificial intelligence and automotive engineering has ushered in a transformative era for mobility. Autonomous vehicles (AVs), once confined to science fiction, are now progressing rapidly toward full-scale deployment. The journey from concept to commercialization has been propelled by the evolution of AI technologies and underpinned by the computational power required to process immense volumes of sensor data in real time. In this domain, NVIDIA stands out not merely as a component supplier, but as an architect of the autonomous driving ecosystem.
Throughout this blog post, we have explored the multi-decade evolution of AVs, the central role of AI in enabling autonomy, and the intricate technical requirements for vehicle perception, localization, planning, and control. NVIDIA’s contribution to this landscape is distinguished by its full-stack approach—an ecosystem that extends from high-performance hardware (like the DRIVE Orin and Thor SoCs) to modular software suites (such as DRIVE OS and DRIVE AV), and into the realm of high-fidelity simulation with Omniverse and DRIVE Sim.
What sets NVIDIA apart is its ability to create end-to-end solutions that are both vertically integrated and horizontally scalable. This enables original equipment manufacturers (OEMs), mobility startups, logistics firms, and governments to customize and deploy AV technologies with reduced development time and greater reliability. The company's success is evident in its extensive partnership network, its adoption across multiple industries, and its leading market share in autonomous vehicle computing platforms.
Looking ahead, the trajectory of AV development will likely be shaped by several key factors:
- Advancements in Generative AI: With the introduction of Transformer Engines in NVIDIA DRIVE Thor, the ability of AVs to understand complex scenarios, generate adaptive responses, and learn continuously from new data will expand significantly.
- Regulatory and Ethical Governance: As AVs become more prevalent, regulatory bodies will require greater transparency and accountability in AI decision-making. NVIDIA’s emphasis on explainable AI and functional safety positions it well to support these requirements.
- Decentralized and Connected Ecosystems: The future of AVs will also depend on vehicle-to-everything (V2X) communication and edge AI capabilities. By integrating connectivity with on-board intelligence, NVIDIA is enabling real-time responsiveness and improved safety in multi-agent environments.
- Sustainability and Smart Infrastructure: AVs are poised to reduce carbon emissions by optimizing routes, improving traffic flow, and integrating with electric vehicle (EV) systems. NVIDIA’s role in building digital twins and supporting smart city infrastructure further enhances its relevance in sustainable urban planning.
In conclusion, autonomous vehicle development is not simply a technological milestone—it represents a reimagining of mobility, safety, and urban life. NVIDIA, through its robust AI platforms and strategic vision, continues to be at the forefront of this evolution. As industries converge on this new frontier, NVIDIA’s end-to-end AV technology stack will likely serve as a cornerstone in building the future of intelligent transportation.
References
- NVIDIA Autonomous Vehicle Solutions
https://www.nvidia.com/en-us/self-driving-cars/ - Waymo Official Blog – Technology
https://blog.waymo.com - Tesla Autopilot and Full Self-Driving Overview
https://www.tesla.com/autopilot - All-Electric, Self-Driving Ridehail Service
https://www.getcruise.com - TuSimple Autonomous Freight Network
https://www.tusimple.com - Mobileye (Intel) Autonomous Driving Tech
https://www.mobileye.com - A Different Kind of Autonomous Vehicle
https://zoox.com - Pony.ai Robotaxi and Freight Technology
https://www.pony.ai - Simulation for Autonomous Vehicles
https://developer.nvidia.com/drive/drive-sim - NVIDIA Omniverse for Automotive and AV Digital Twins
https://developer.nvidia.com/nvidia-omniverse